课件地址:https://github.com/wangshusen/RecommenderSystem
结合小红书的业务场景和内部实践,讲解主流的工业界推荐系统技术。
主要内容:
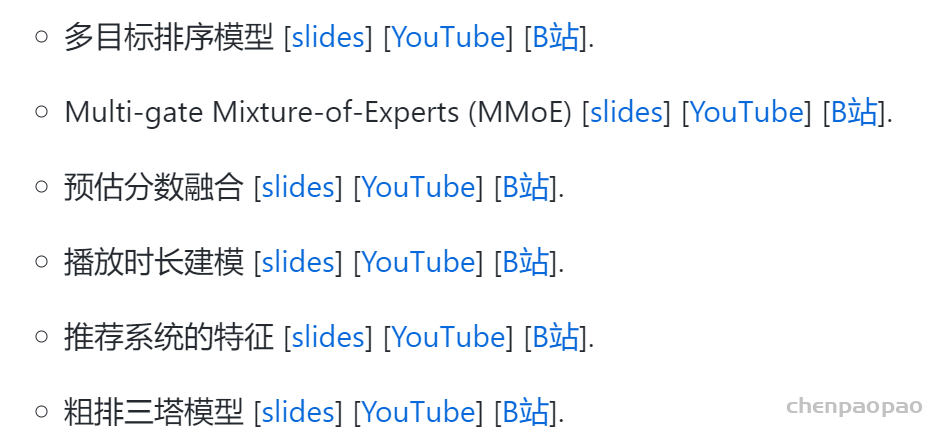
排序01:多目标模型
第一部分是模型结构。模型把用户特征、物品特征、统计特征、场景特征作为输入,输出对多个指标的预估。 第二部分内容是降采样和校准。在实际的推荐系统中,正负样本的比例严重不平衡,负样本数量远多于正样本,因此需要对负样本做降采样。以点击率为例,对负样本做降采样会导致模型高估点击率,因此需要用公式做校准。
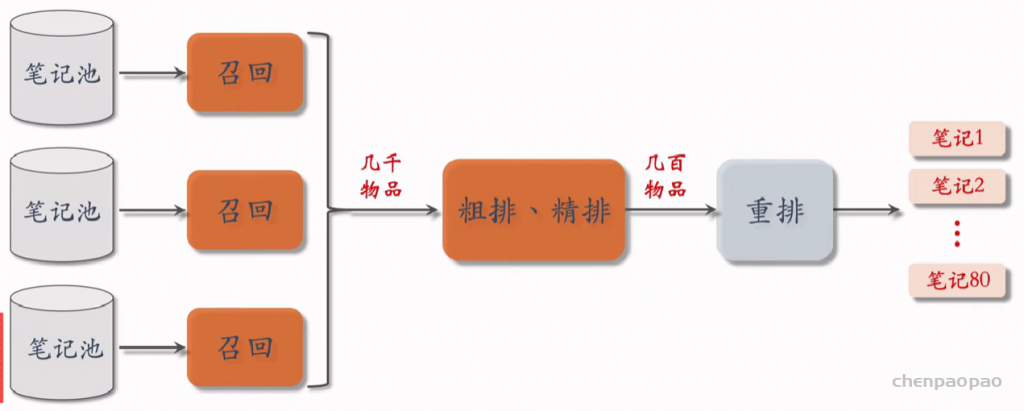
粗排和精排用到的方法都差不多,但精排的模型比较大,因此需要先用粗排模型从几千篇笔记中选择几百篇用于精排。
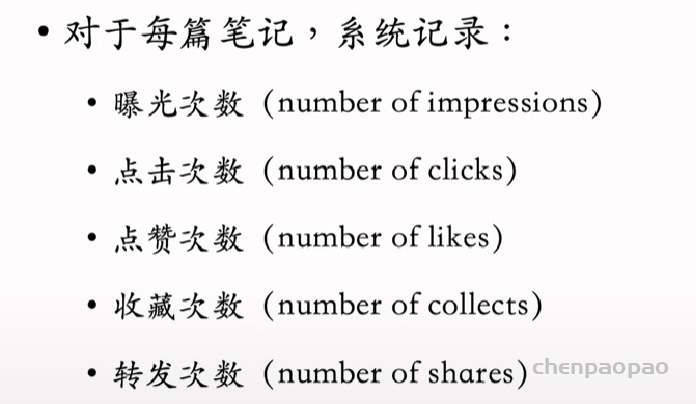
用户点击以后才会出现点赞、收藏和转发行为:
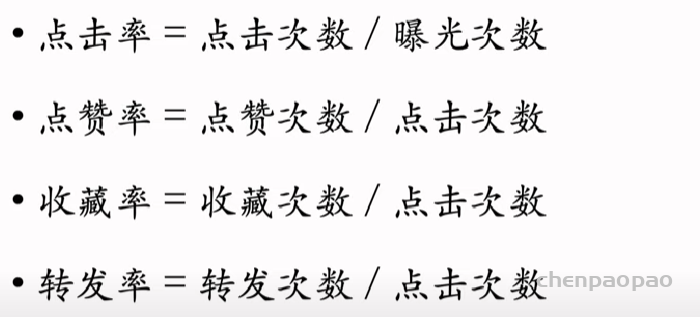
排序的依据是笔记的点击率、点赞率、收藏率、转发率等多种分数。
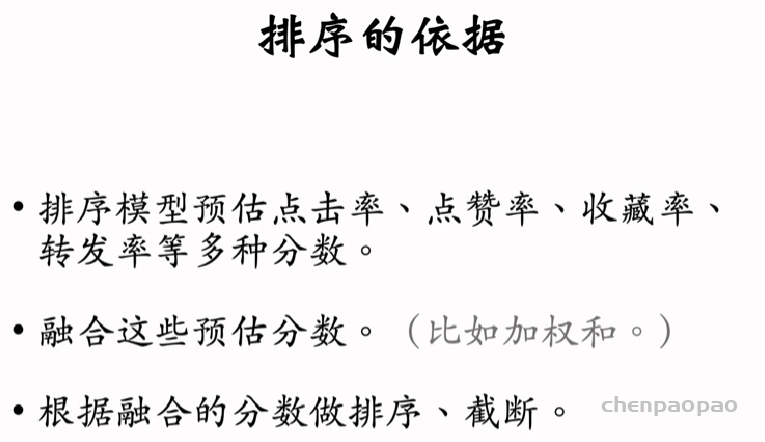
多目标模型:
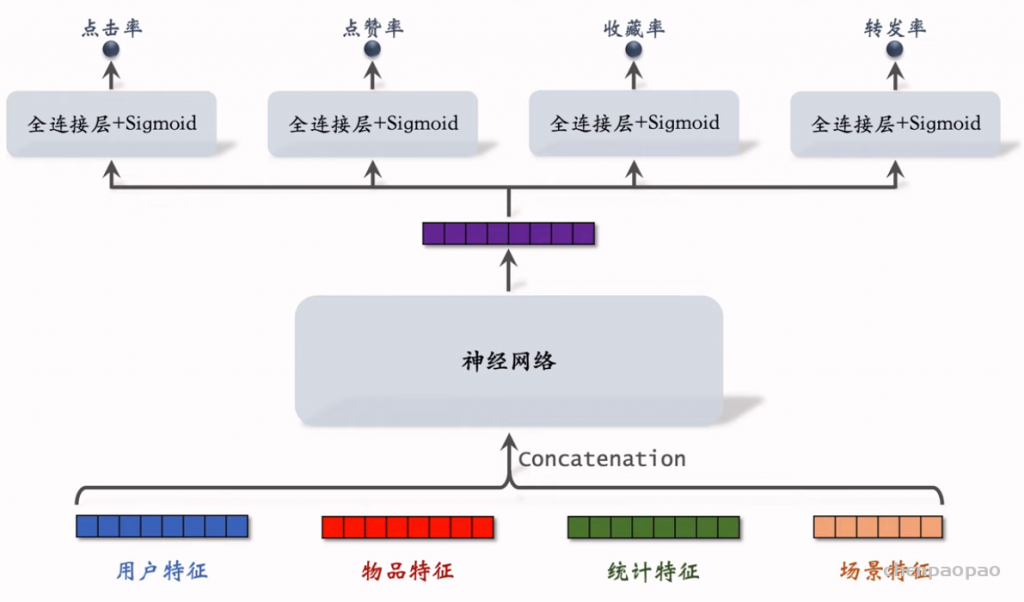
模型输出某个用户对于某篇笔记的预估点赞率、点击率、收藏率以及转发率。
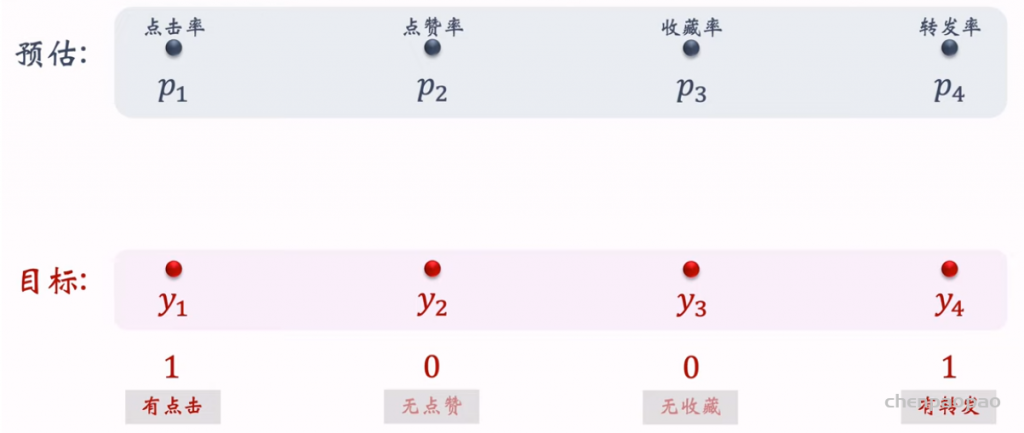
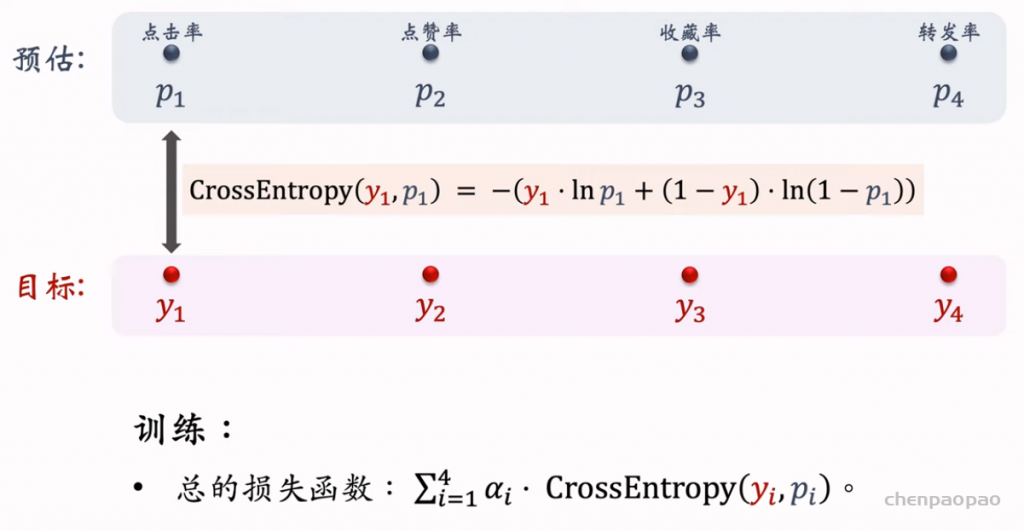
训练:类别不平衡。
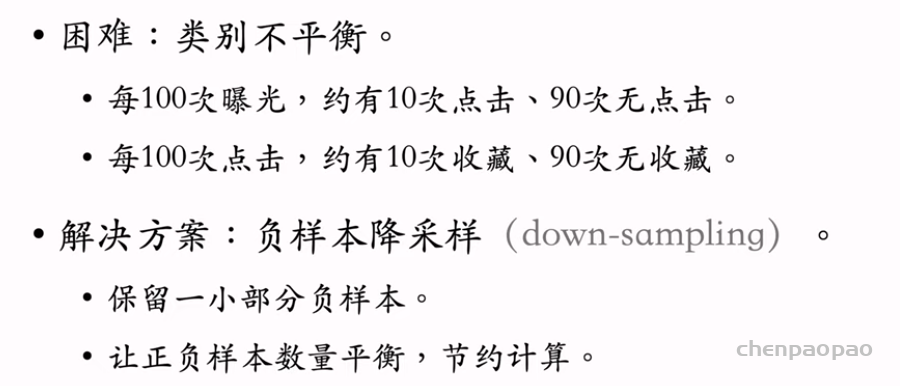
预估值校准
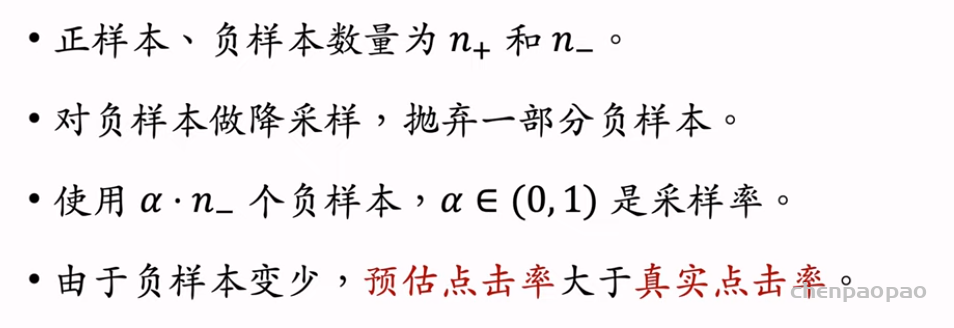
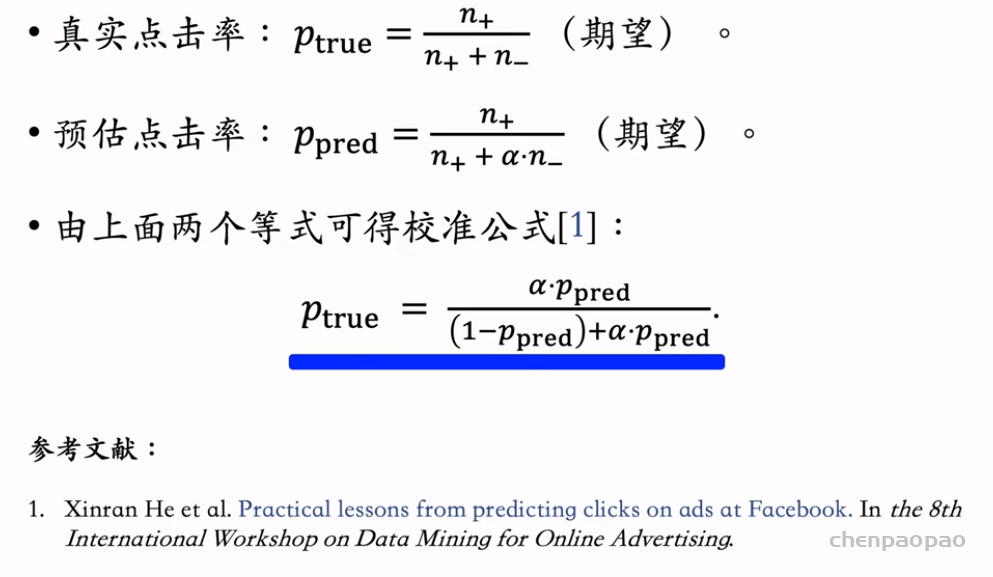
排序02:多目标排序模型的改进MMoE
Multi-gate Mixture-of-Experts (MMoE) 是一种多目标排序模型。MMoE 用多个独立的塔提取特征,并对多塔提取的特征向量做加权平均,然后送入多头。MMoE 的训练容易出现极化现象(polarize),可以用 dropout 解决。
这里假设神经网络的目标只有点击率和点赞率这两个目标,因此只需要两组权重:p和q
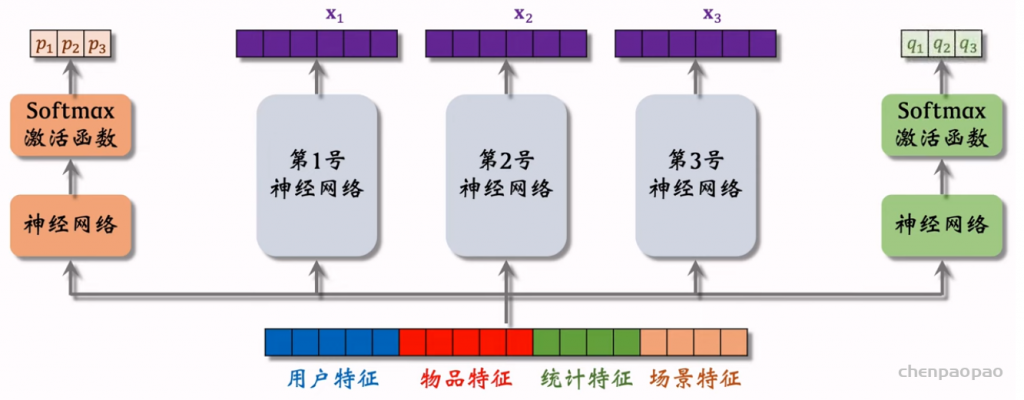
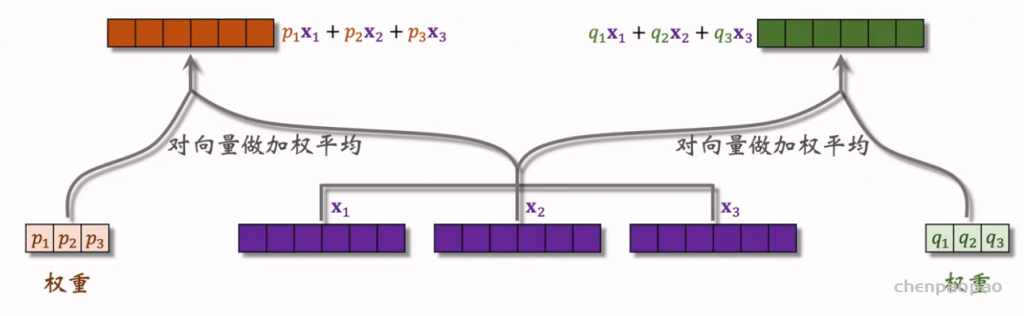
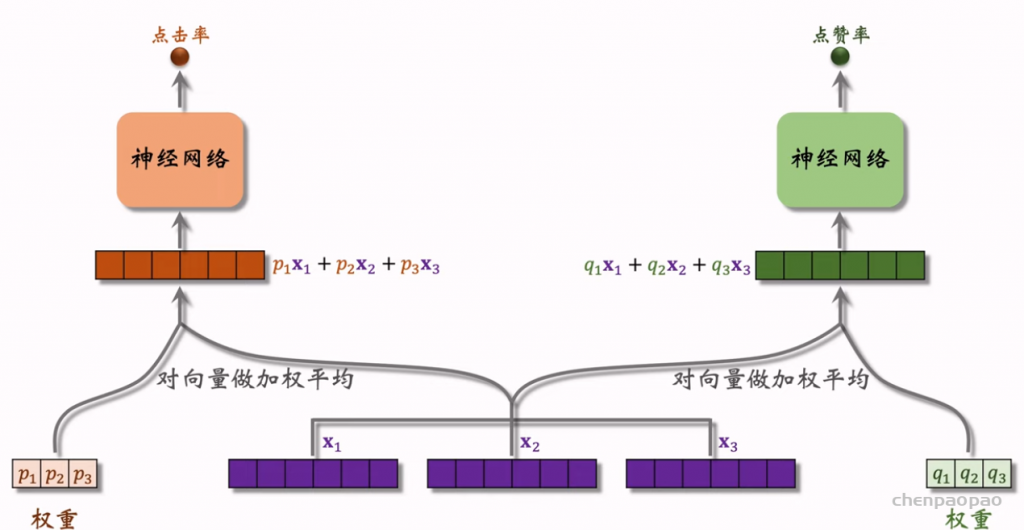
极化现象:softmax函数导致
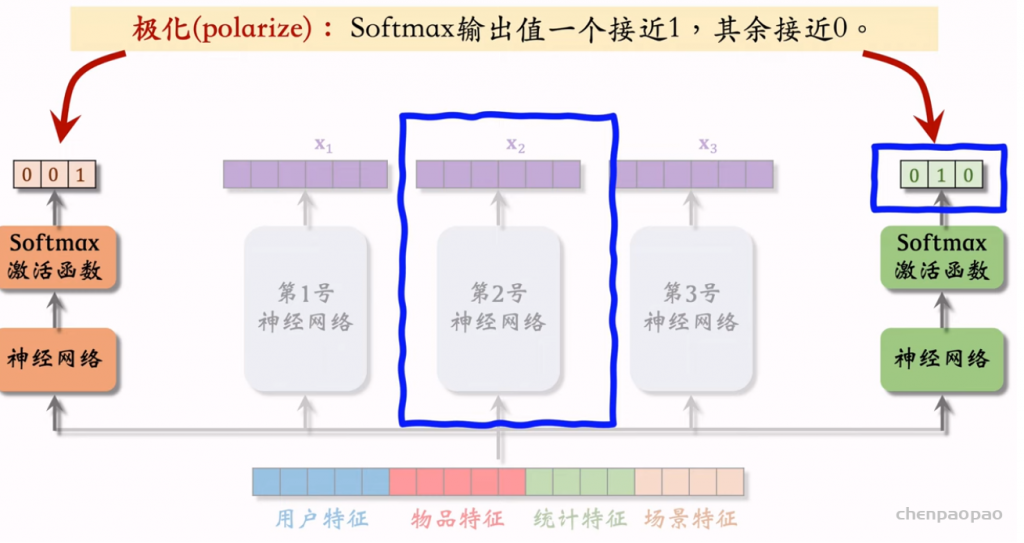
极化解决方法:
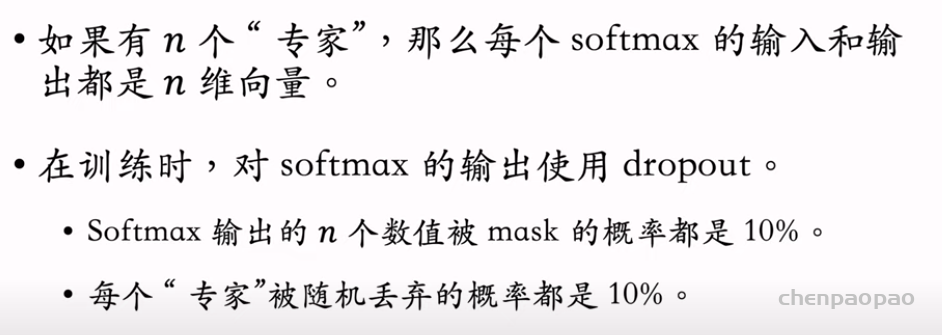
排序03:预估分数的融合
前面通过MMoE获得了点赞、点击、转发率等指标的预估值,得到这些指标之后,需要将它们融合成一个分数。这节课介绍工业界常用的几种融分公式。
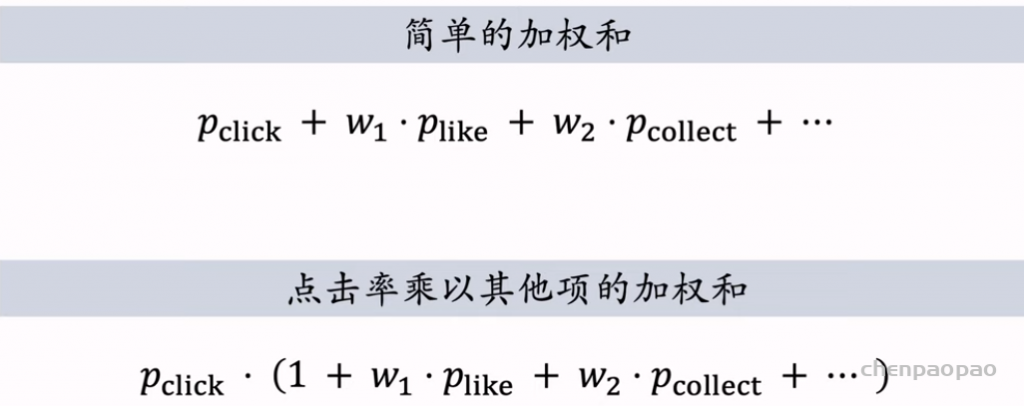
用预估的视频时长做排序,用排名来计算得分:
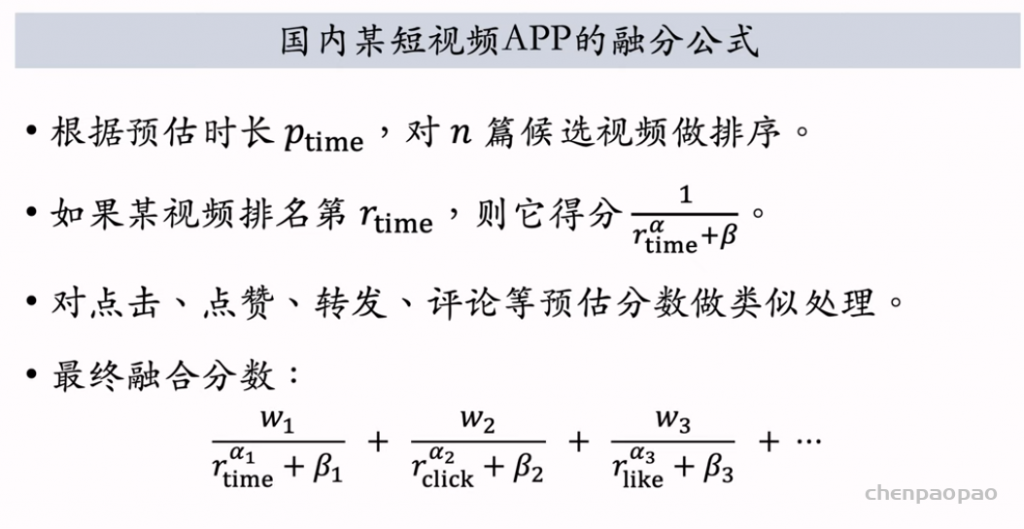
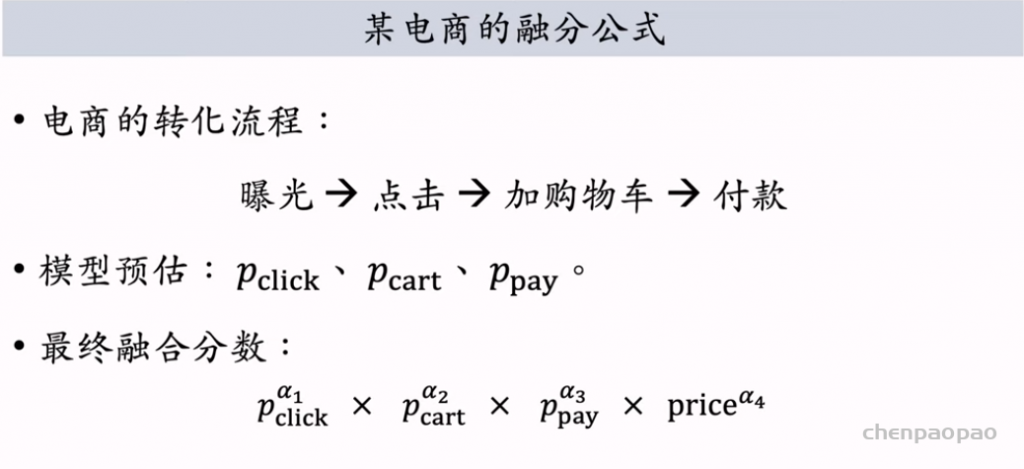
排序04:视频播放建模
视频播放的建模主要有两个目标:视频播放时长、视频完播率。这节课讨论如何在多目标排序模型添加与播放时长、完播率相关的预估任务。
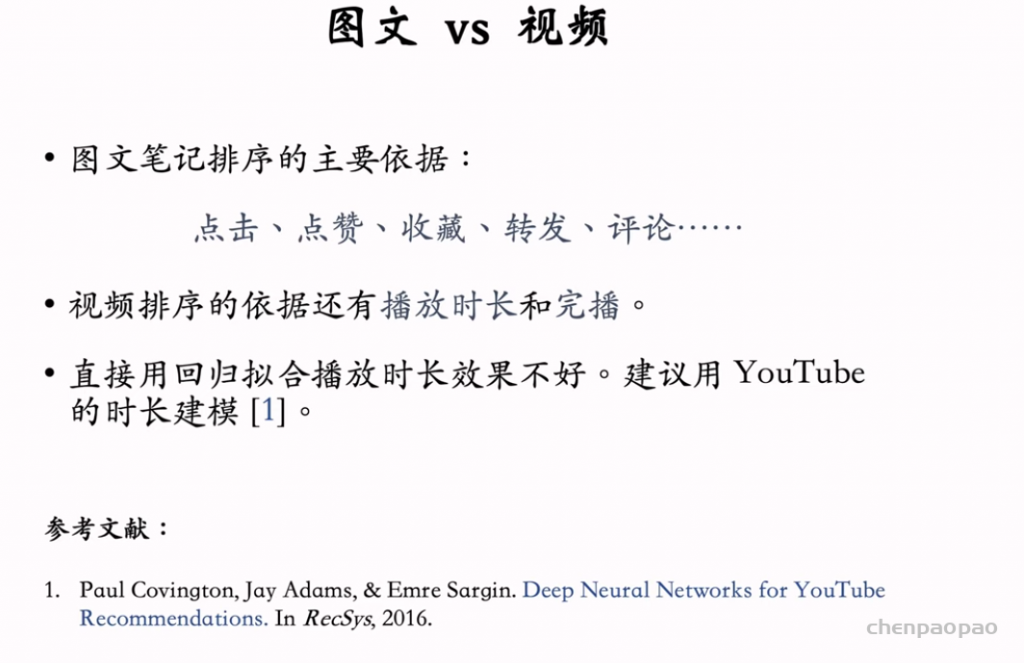
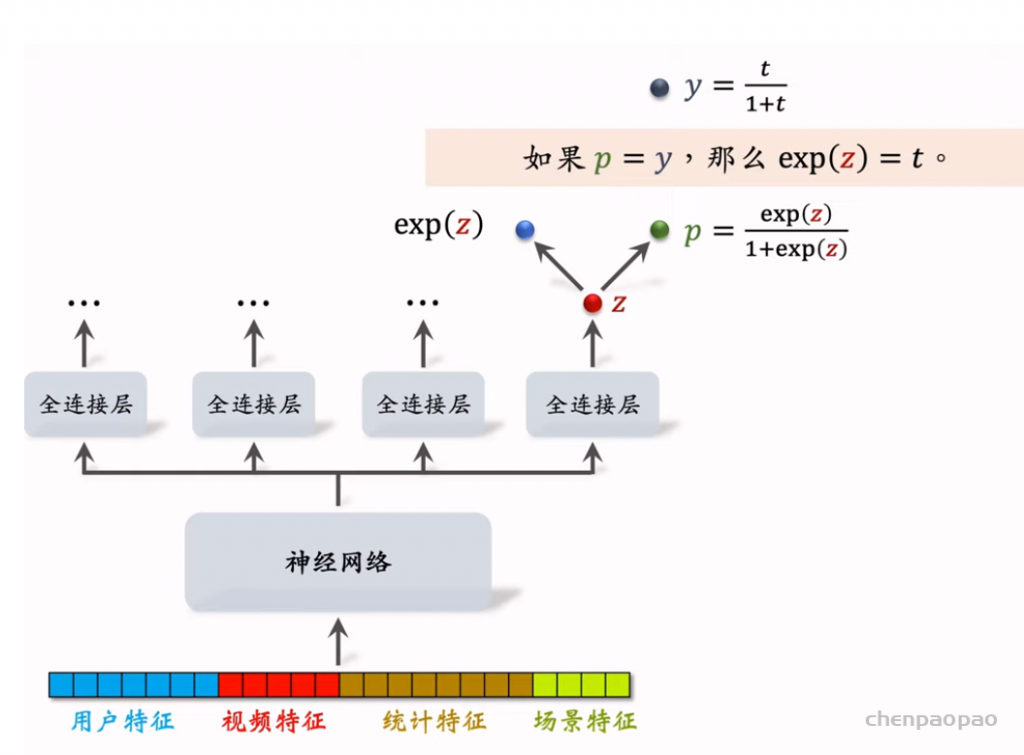
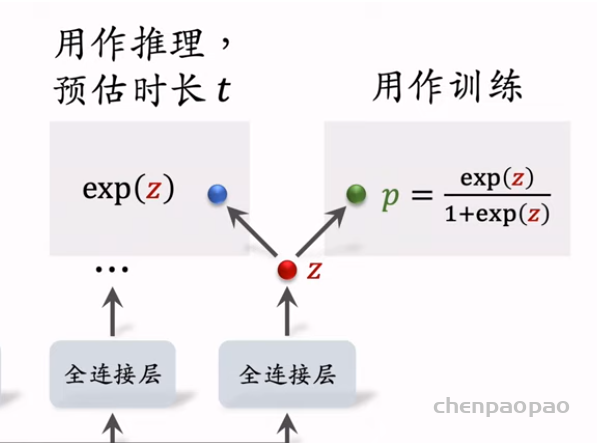
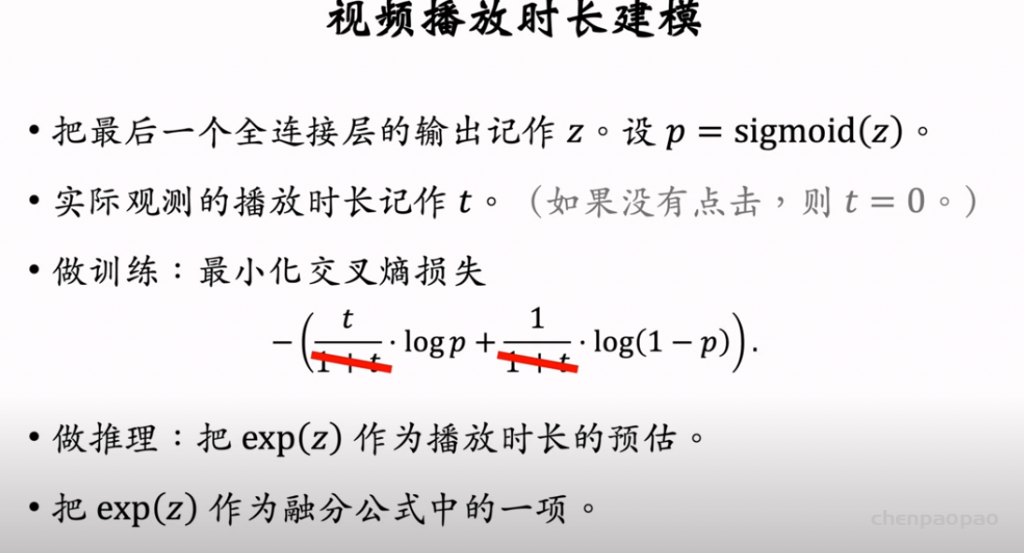
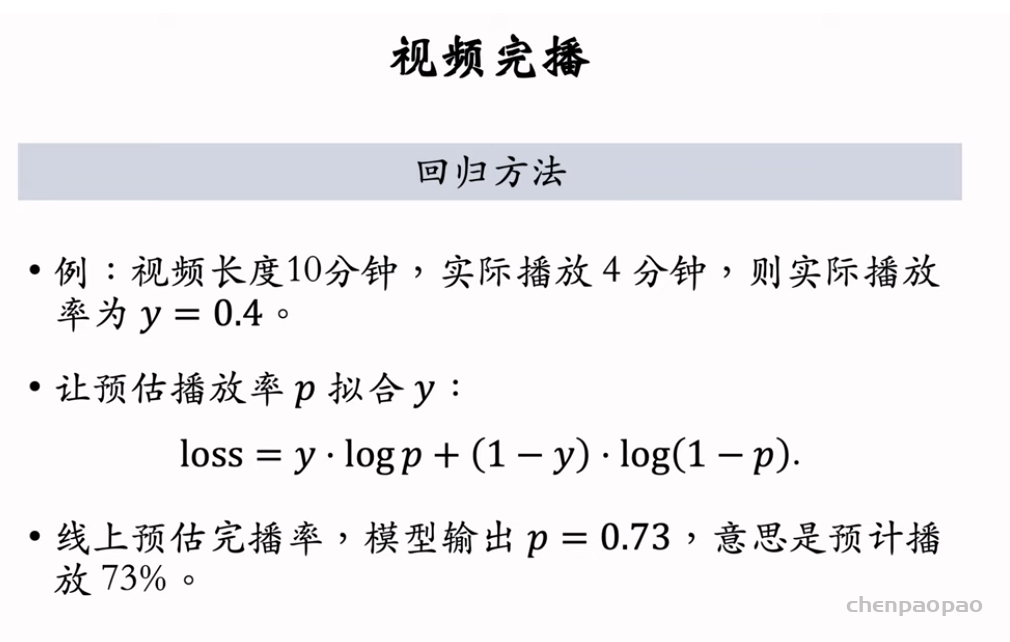
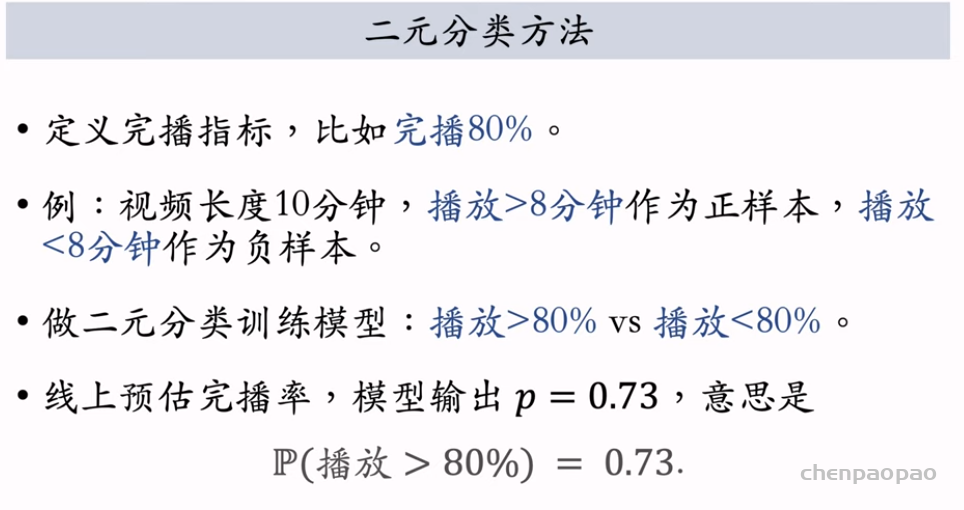
视频越长,完播率越低:
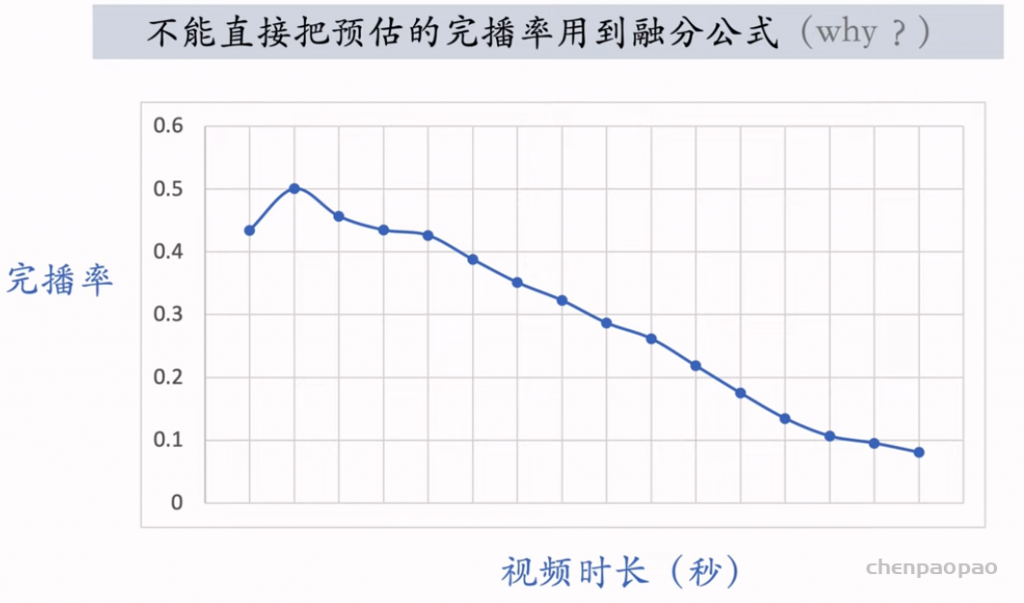
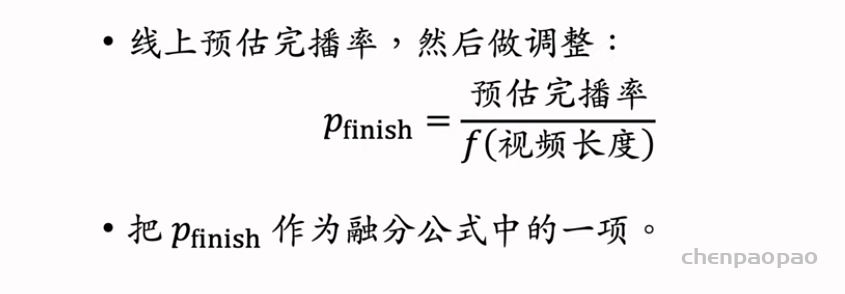
排序05:排序模型的特征
推荐系统中的排序模型需要用户画像、物品画像、统计特征、场景特征。
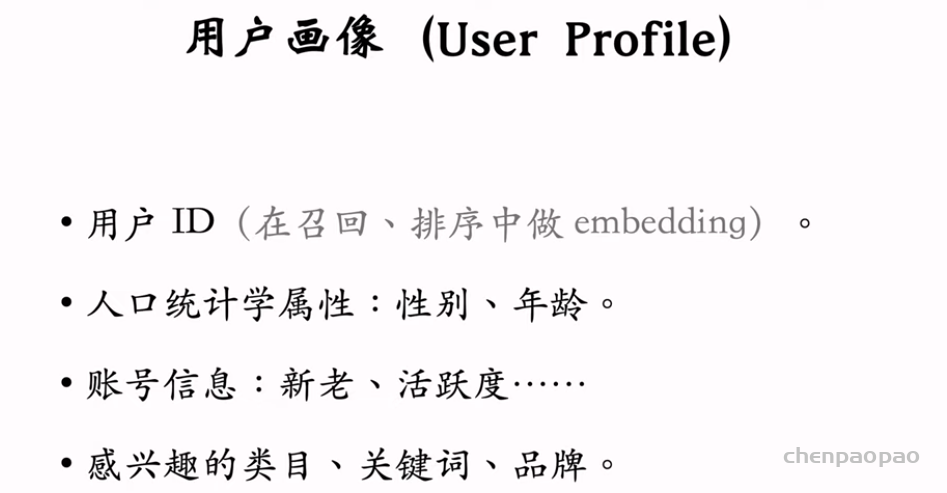
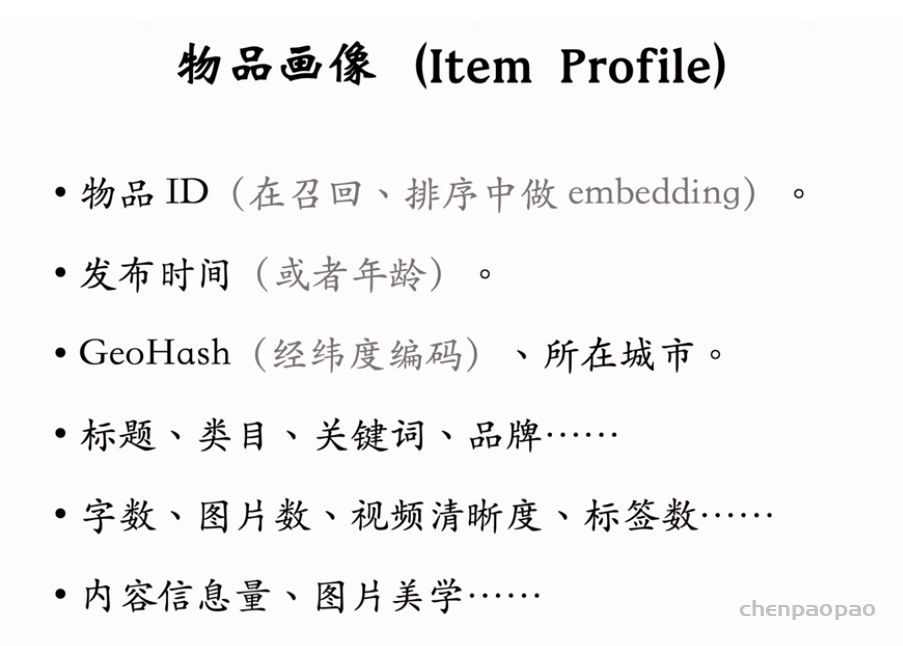
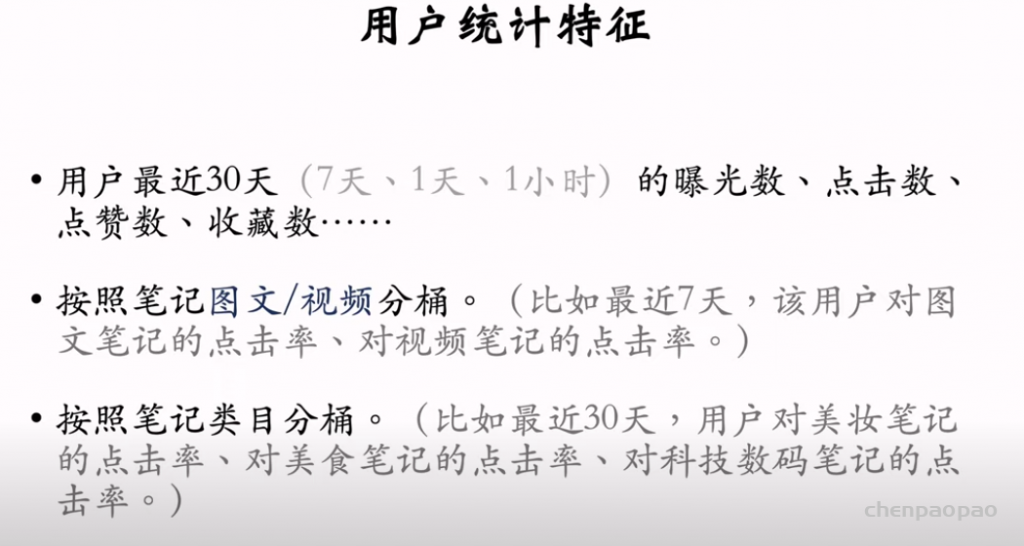
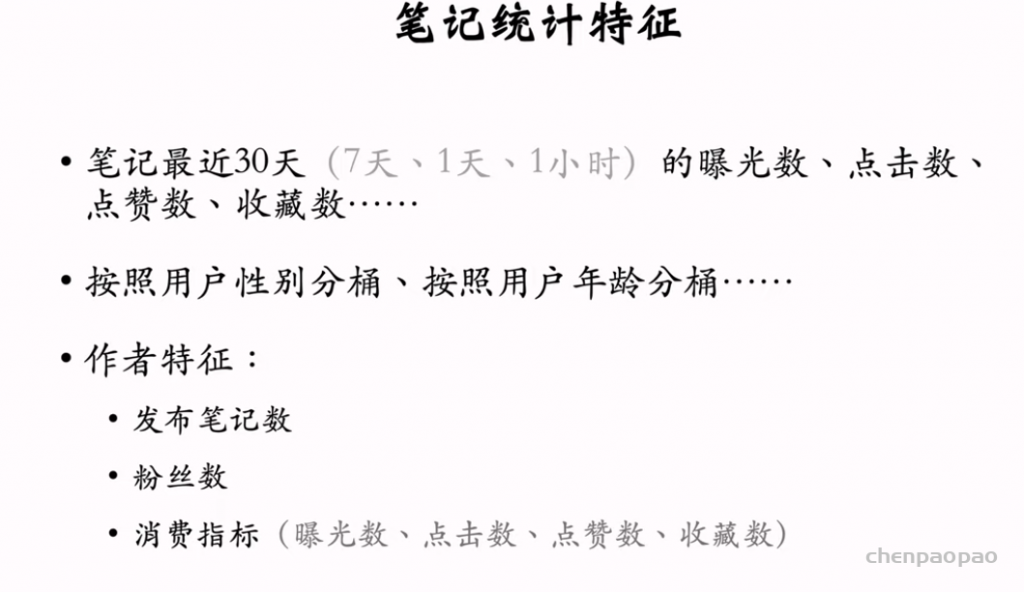
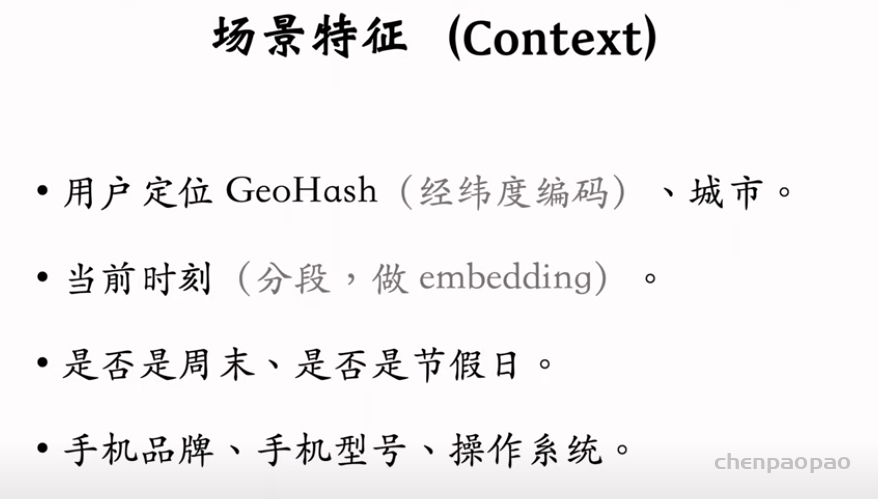
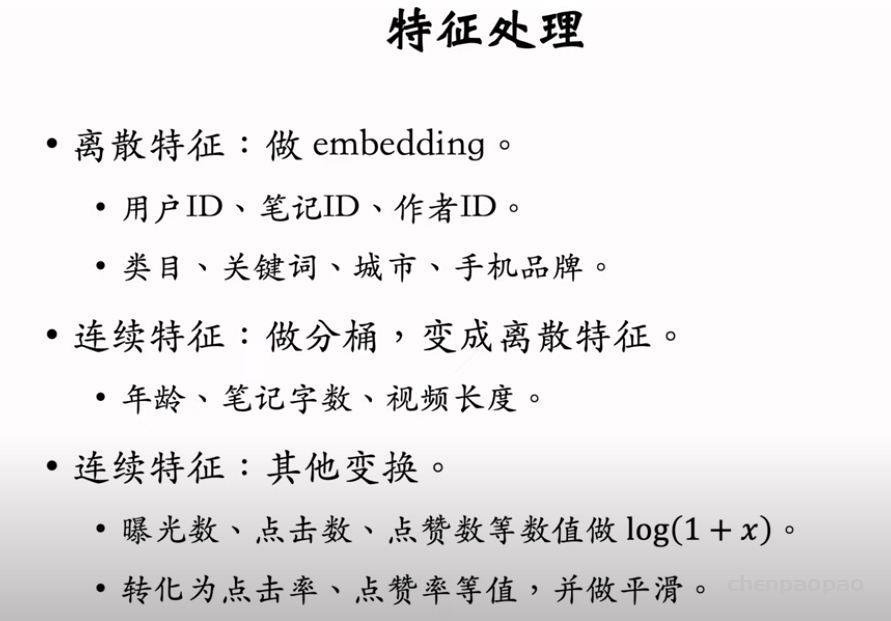
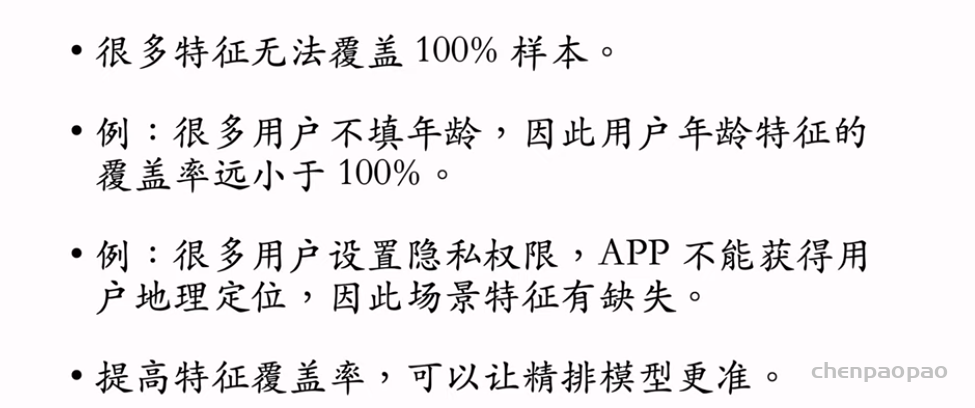
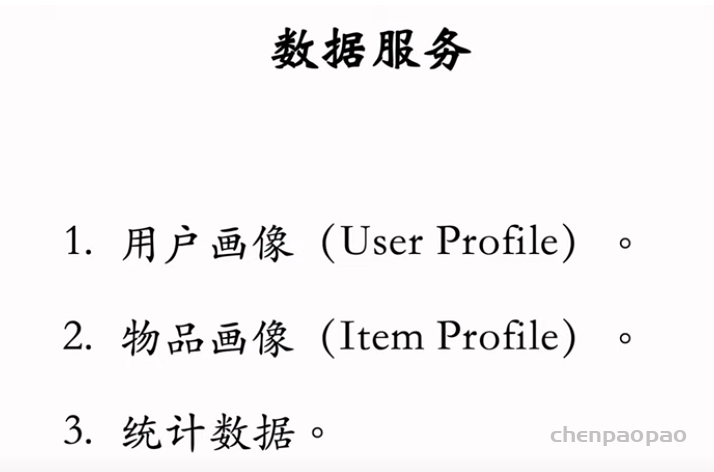
排序06:粗排的三塔模型
粗排三塔模型,它介于前期融合的双塔模型和后期融合的精排模型之间。用户塔可以很大,因为它只需要做1次推理。物品塔可以比较大,因为物品塔的输出可以缓存在PS。交叉塔必须很小,如果有n个物品,交叉塔需要做n次推理。三塔模型的计算量主要是上层的全连接层。
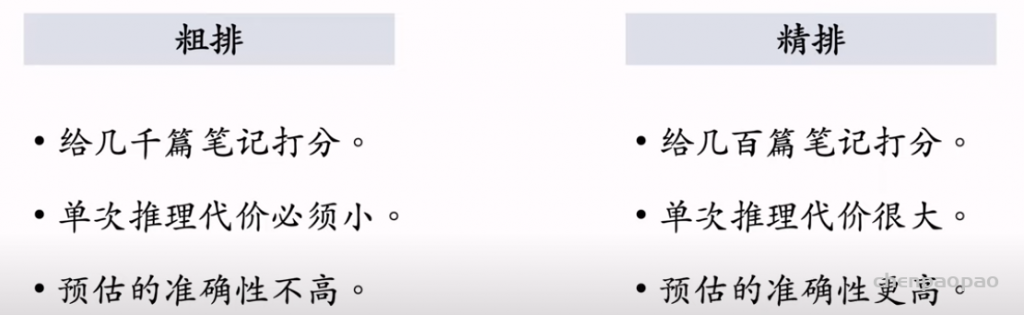
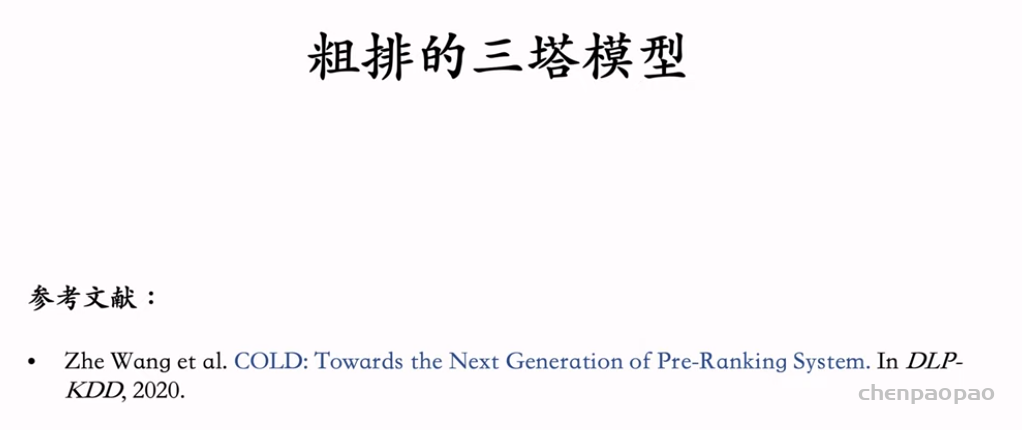
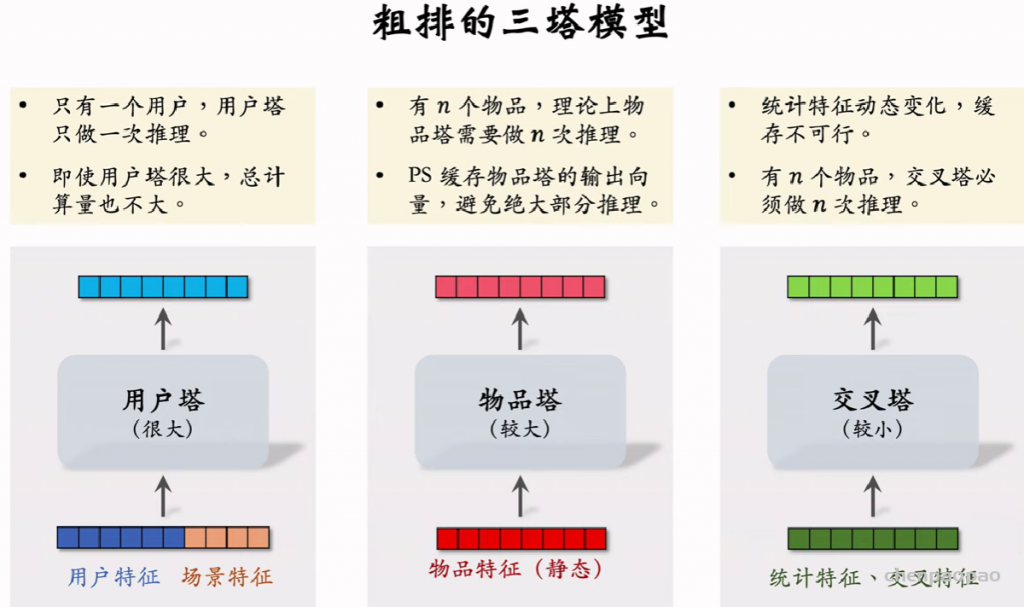
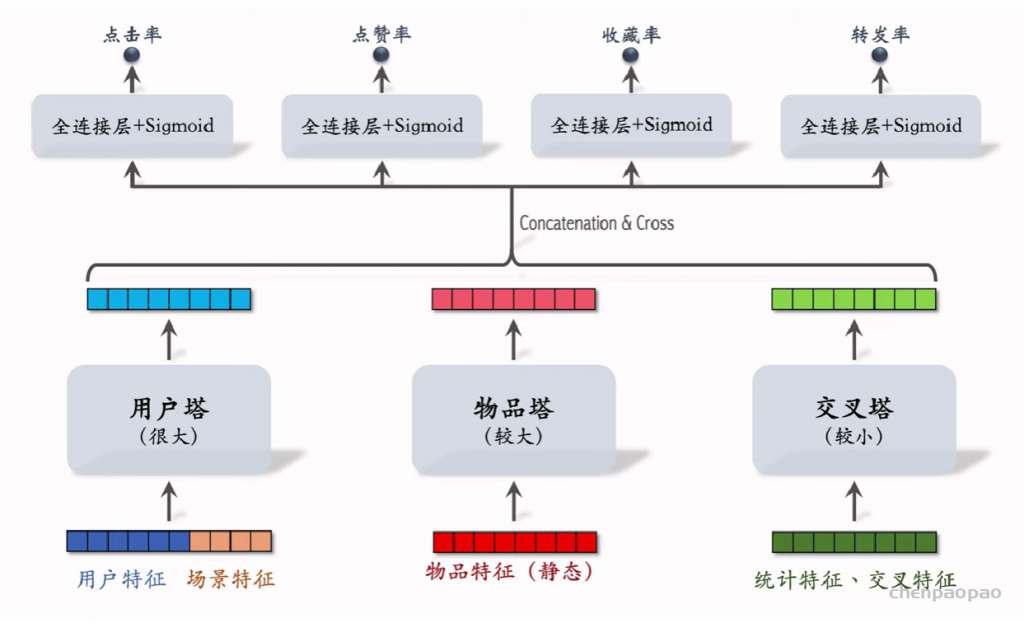
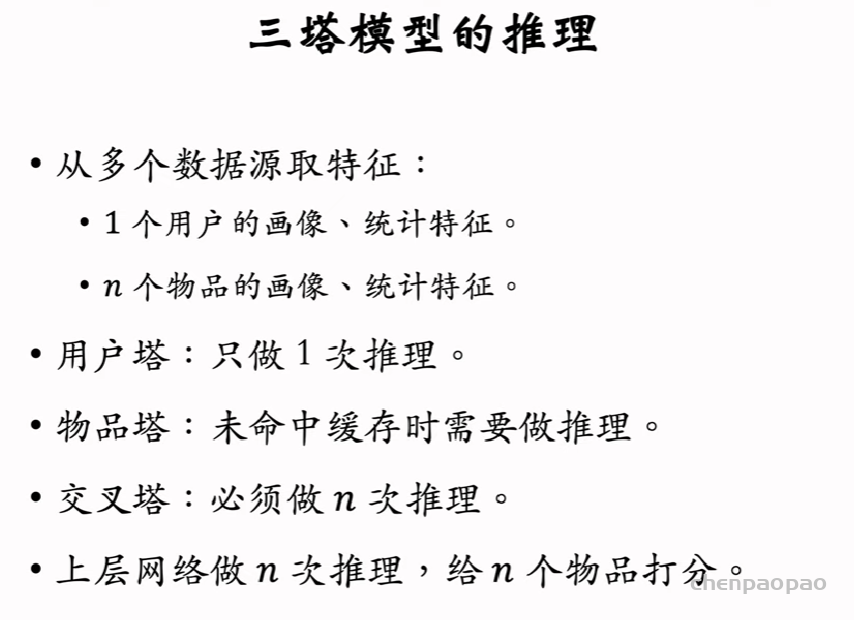