重排01:物品相似性的度量、提升多样性的方法
推荐系统中的多样性。如果多样性做得好,可以显著提升推荐系统的核心业务指标。这节课的内容分两部分: 1. 物品相似性的度量。可以用物品标签或向量表征度量物品的相似性。最好的方法是基于图文内容的向量表征,比如 CLIP 方法。 2. 提升多样性的方法。在推荐的链路上,在粗排和精排的后处理阶段,综合排序模型打分和多样性分数做选择。
为了提升物体的多样性,首先要度量两个物品有多相似。
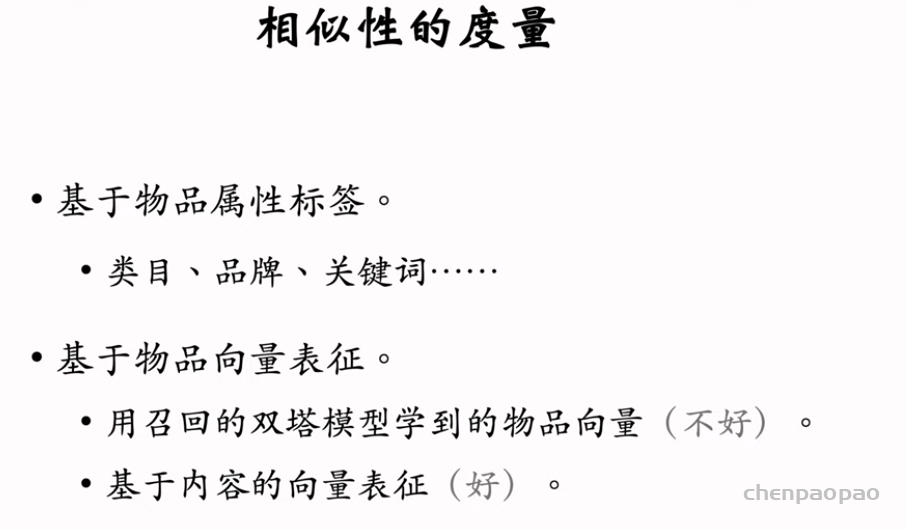
基于物品属性标签计算相似度:
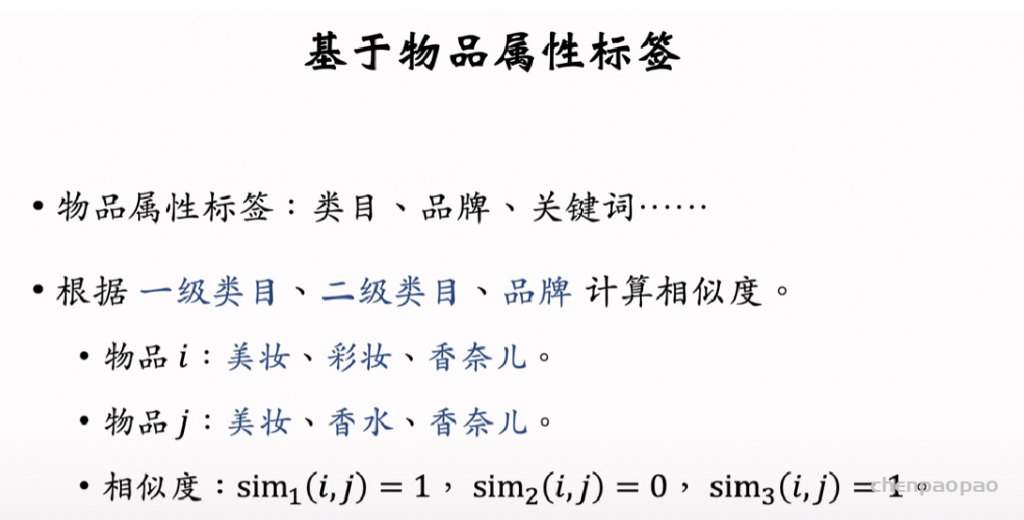
基于物品向量表征:基于图文内容的向量表征
用下面的方法不好训练,因为需要人工标注,因此一般采用cilp的方法。
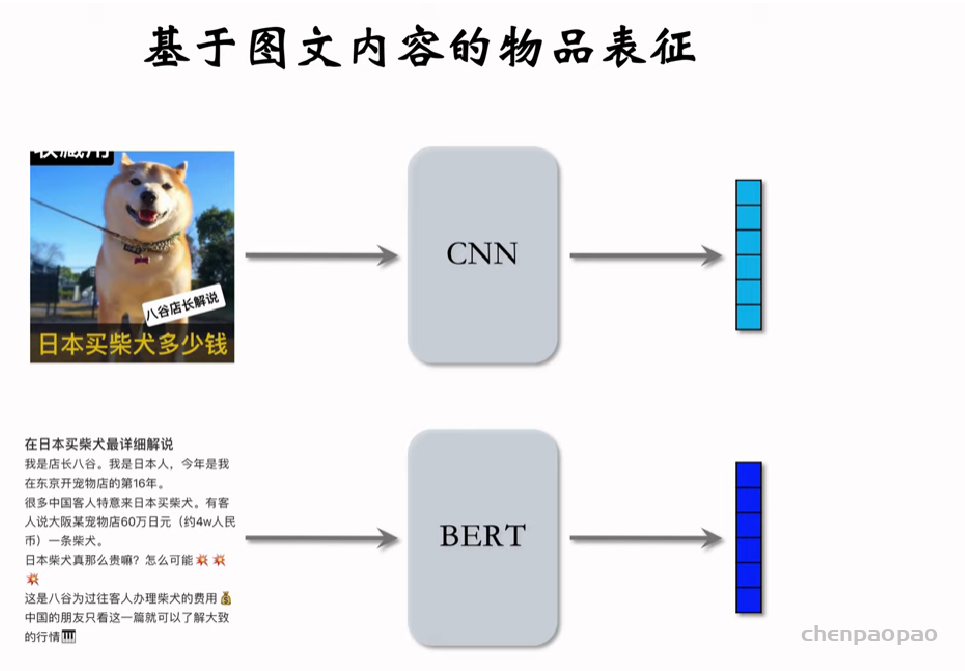
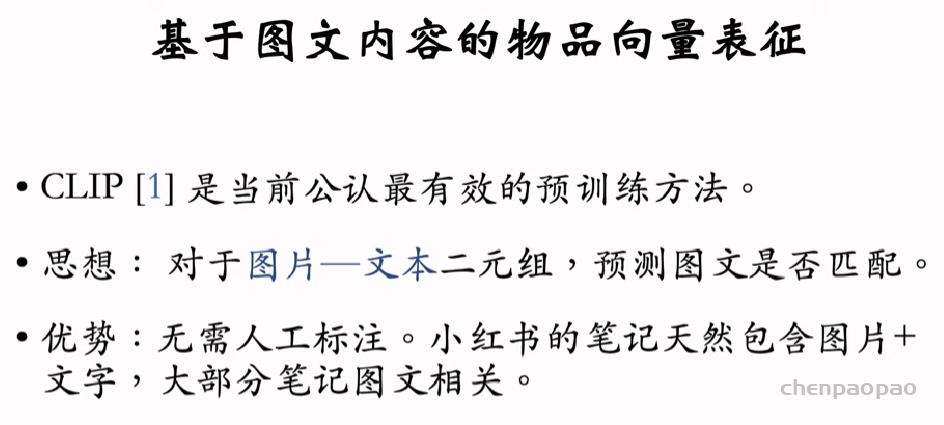
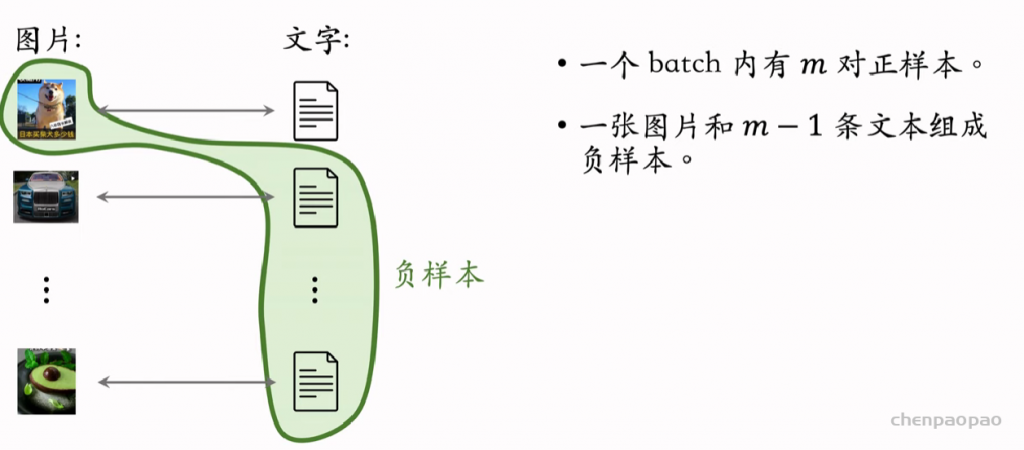
提升多样性的方法:
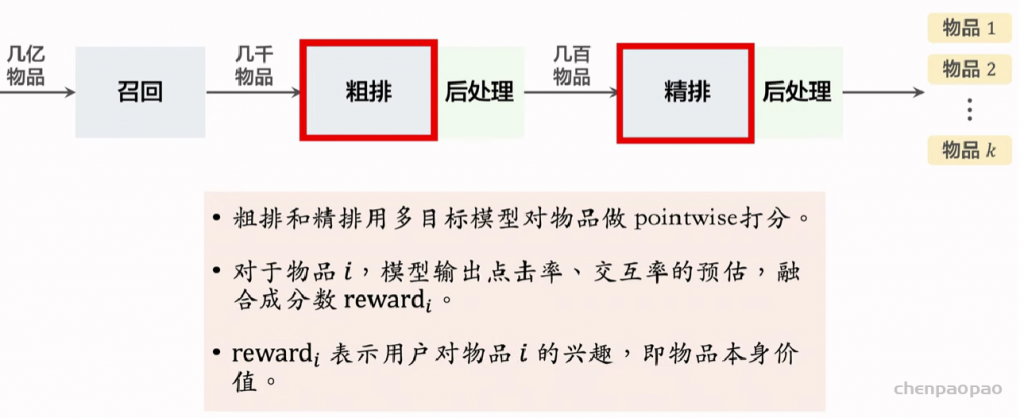
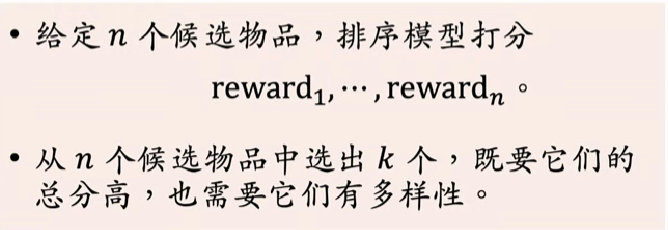
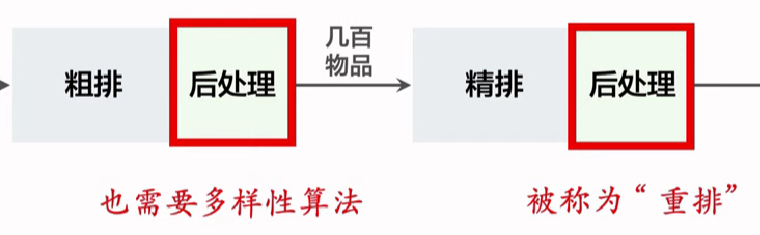
重排02:MMR 多样性算法(Maximal Marginal Relevance)
推荐系统和搜索引擎重排中常用的 Maximal Marginal Relevance (MMR),它根据精排打分和物品相似度,从 n 个物品中选出 k 个价值高、且多样性好的物品。这节课还介绍滑动窗口 (sliding window),它可以与 MMR、DPP 等多样性算法结合,实践中滑动窗口的效果更优。
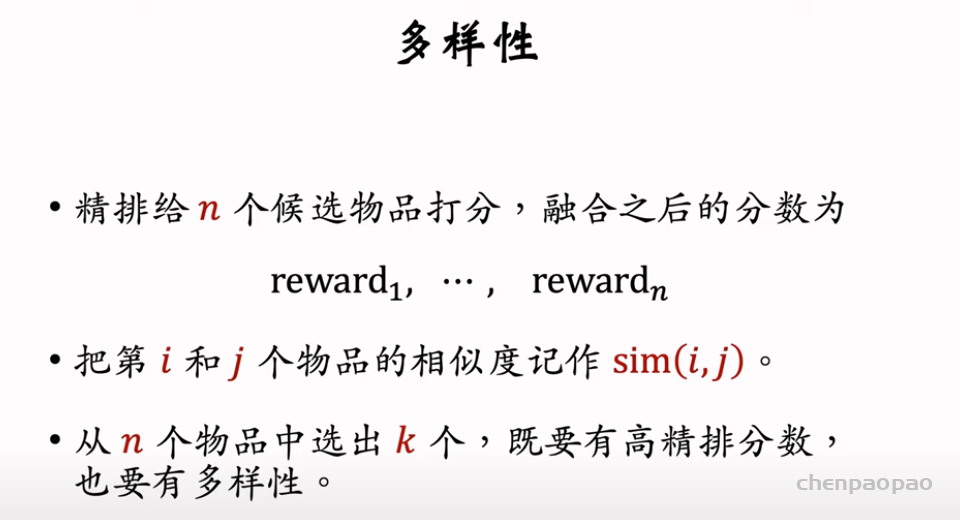
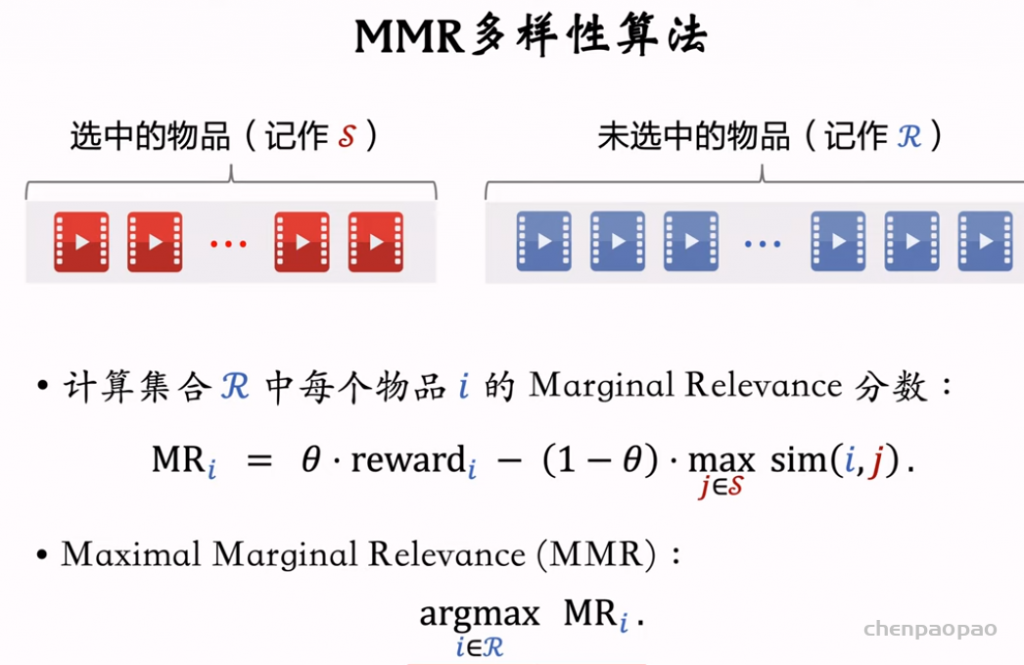
选择R中MR最高的放入集合S中。
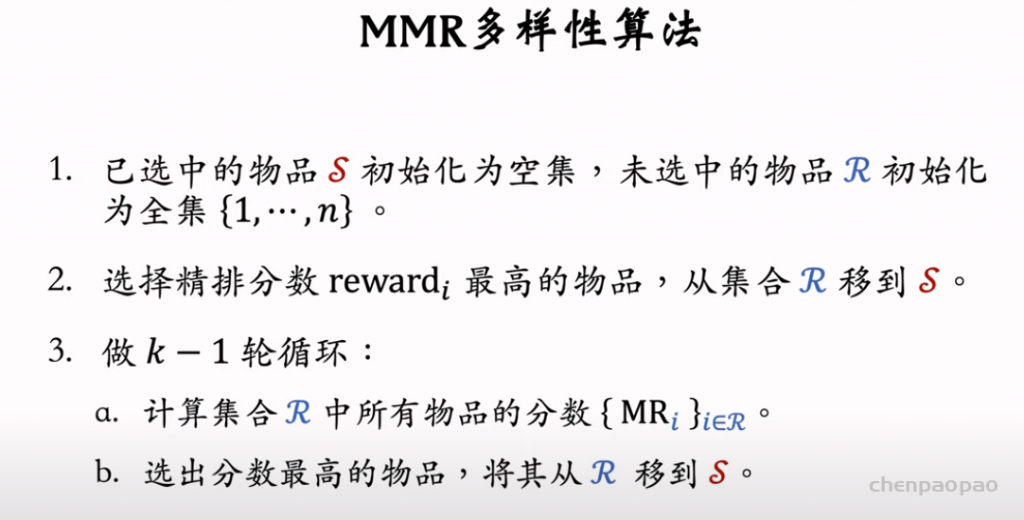
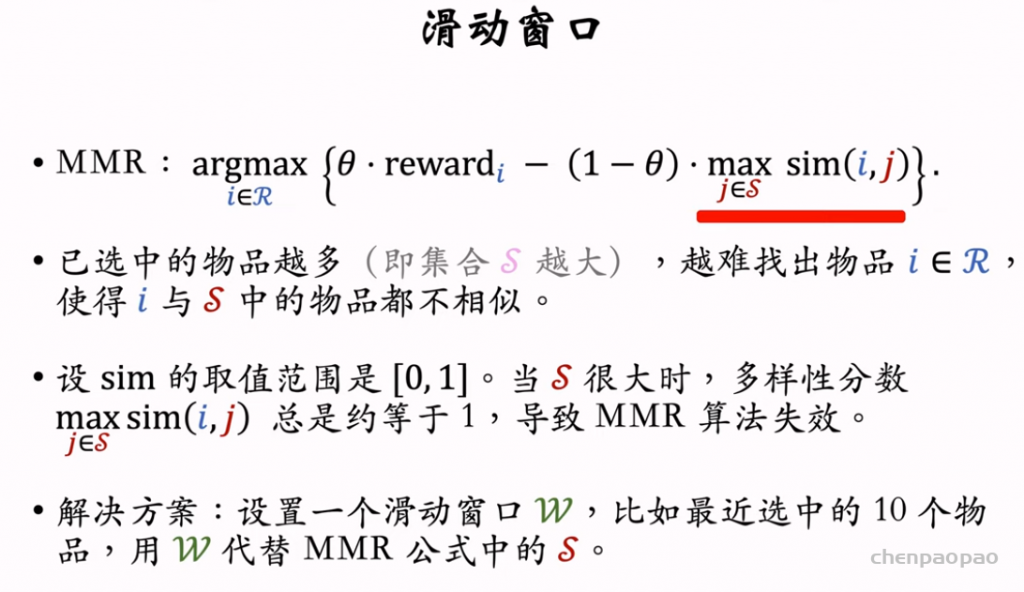
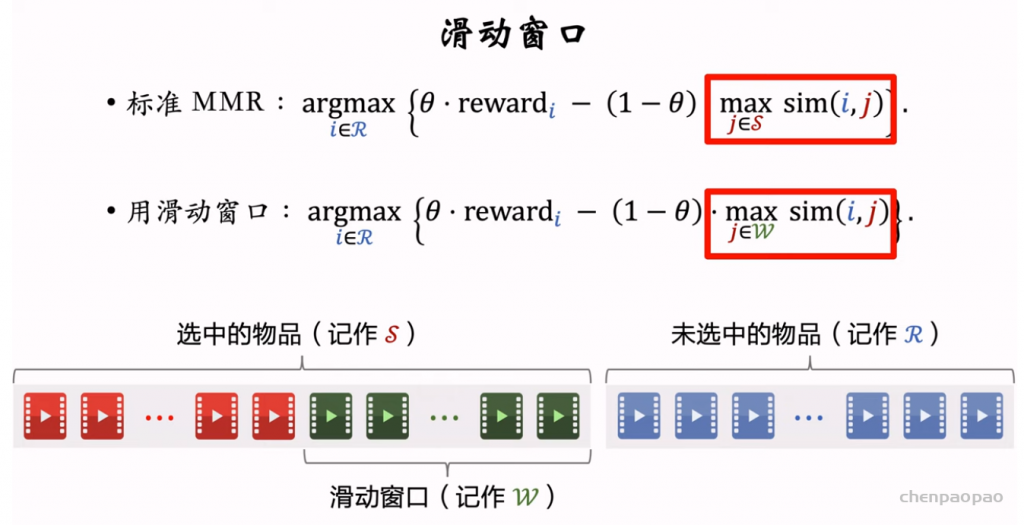
参考文献: Carbonell and Goldstein. The use of MMR, diversity-based reranking for reordering documents and producing summaries. In ACM SIGIR Conference on Research and Development in Information Retrieval, 1998.
重排03:业务规则约束下的多样性算法
推荐系统有很多业务规则,比如不能连续出多篇某种类型的物品、某两种类型的物品笔记间隔多少。这些业务规则应用在重排阶段,可以与 MMR、DPP 等多样性算法相结合。
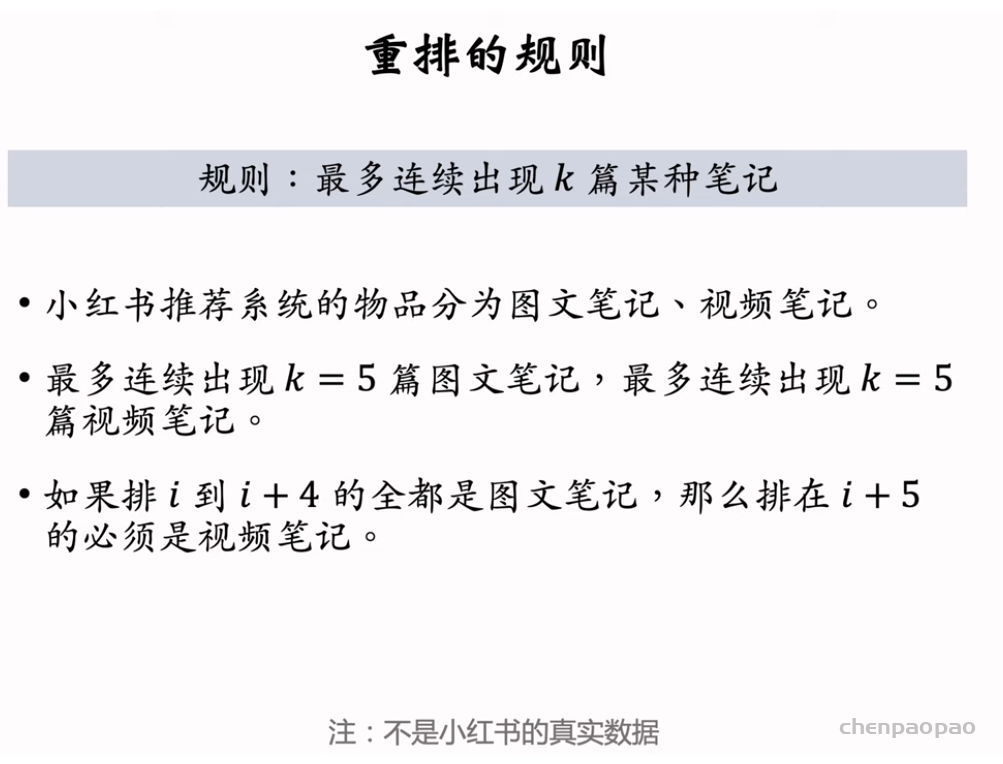
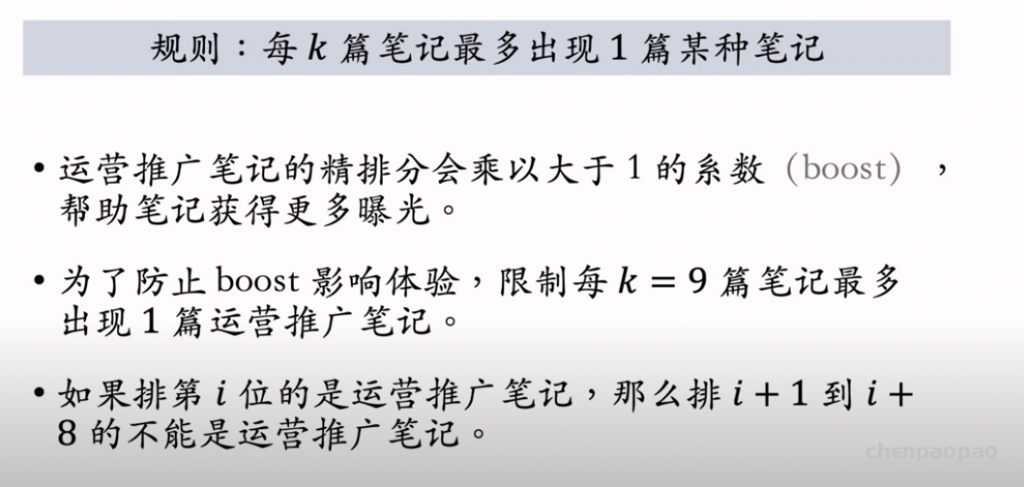
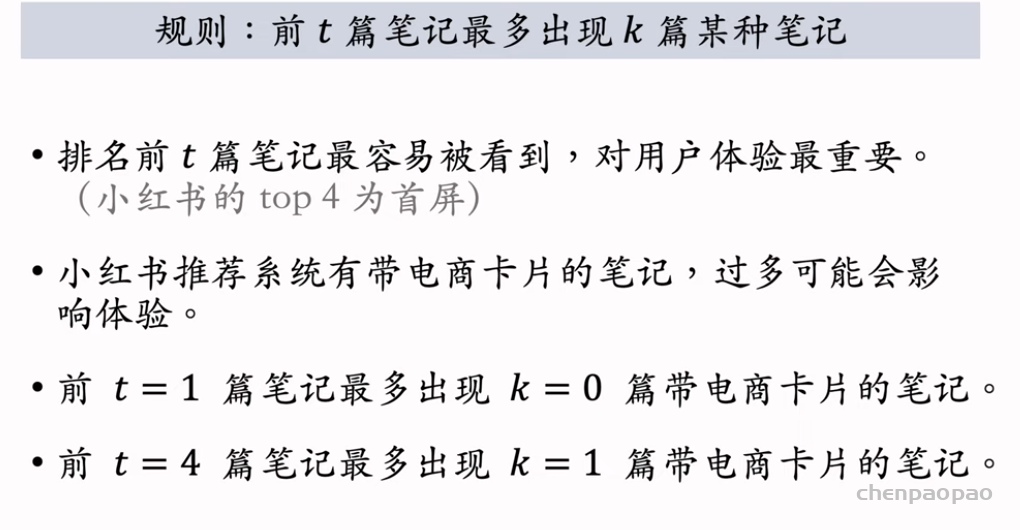
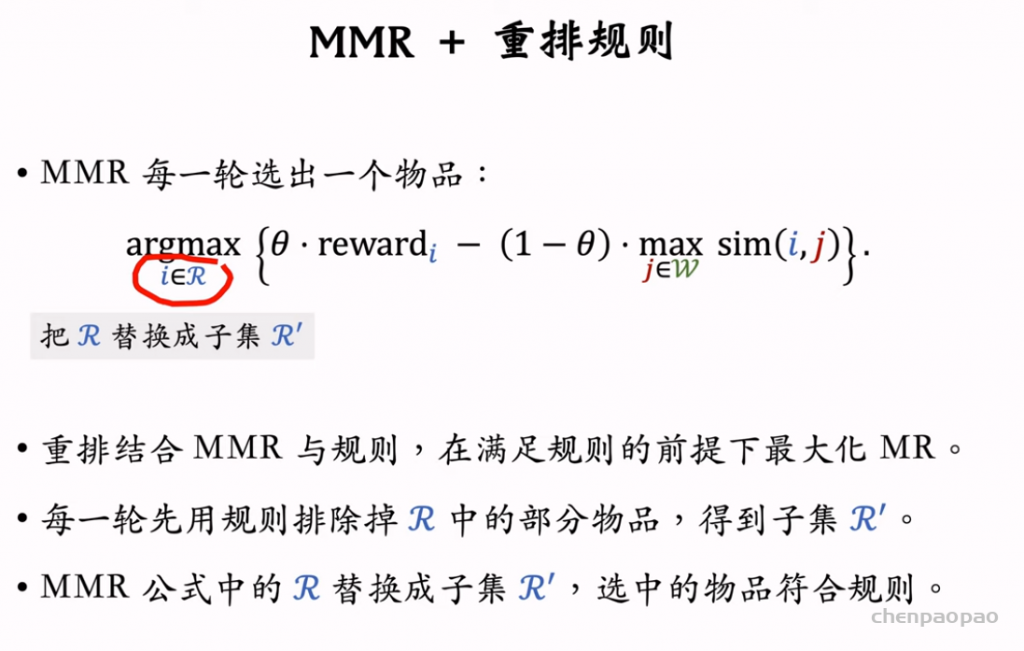
重排04:DPP 多样性算法
行列式点过程 (determinantal point process, DPP) 是一种经典的机器学习方法,在 1970’s 年代提出,在 2000 年之后有快速的发展。DPP 是目前推荐系统重排多样性公认的最好方法。 DPP 的数学比较复杂,内容主要是超平行体、超平行体的体积、行列式与体积的关系。
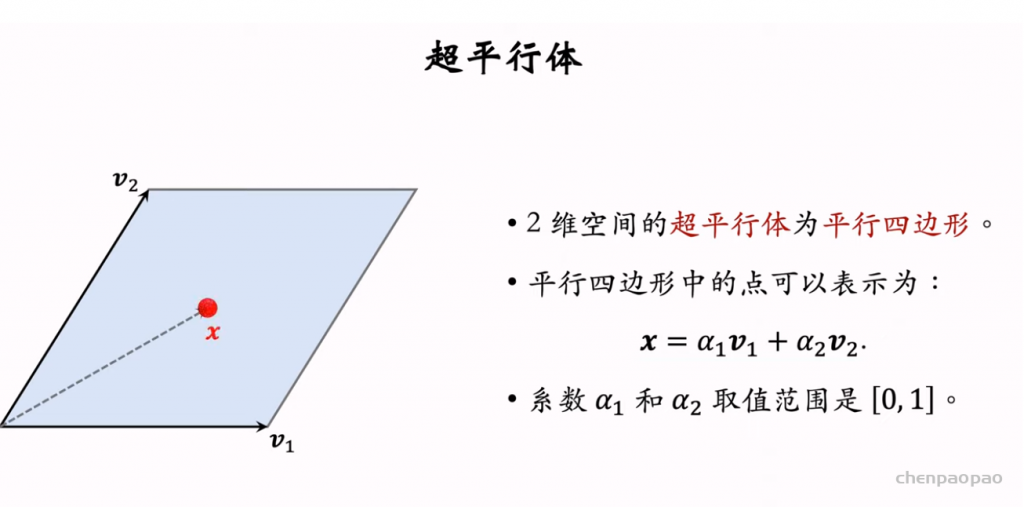
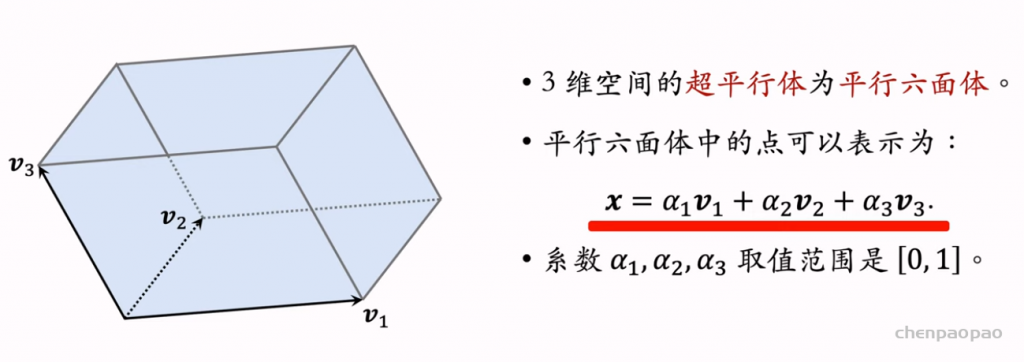
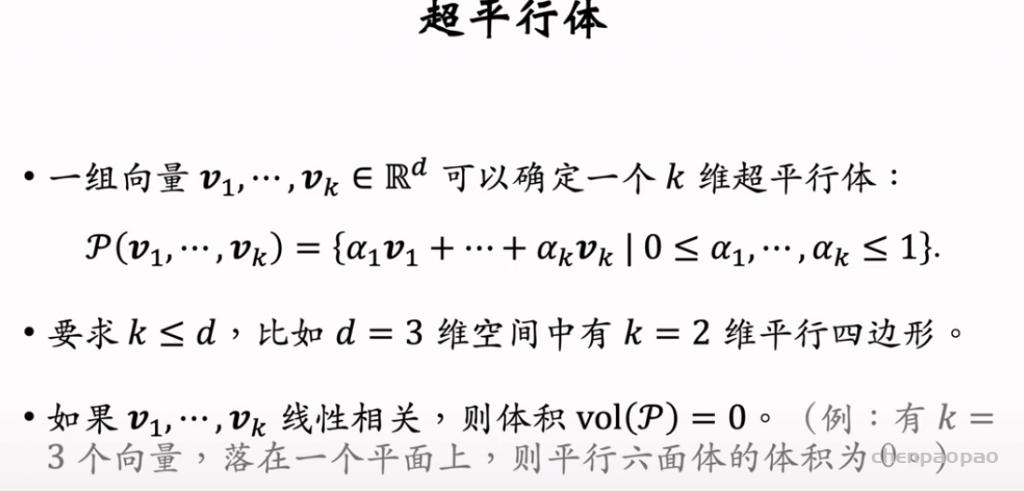
参考文献: Chen et al. Fast greedy map inference for determinantal point process to improve recommendation diversity. In NIPS, 2018.
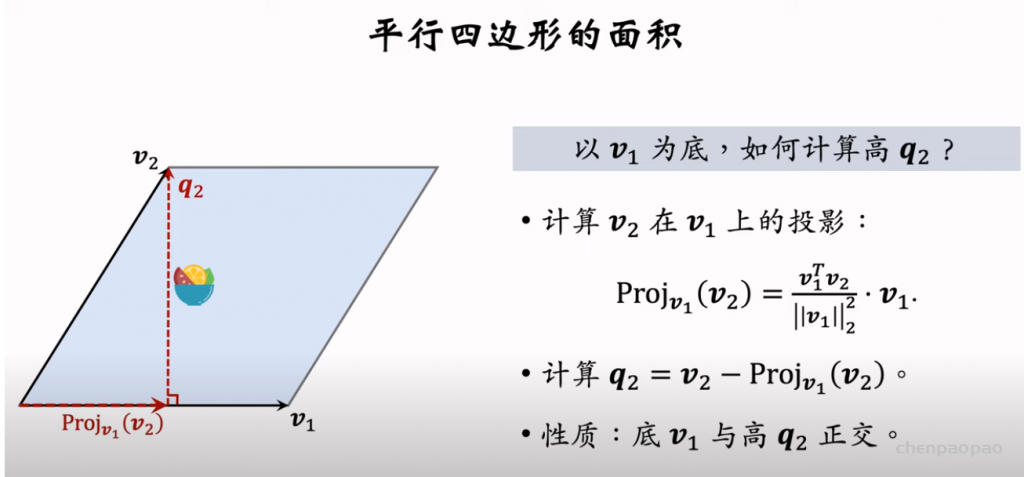
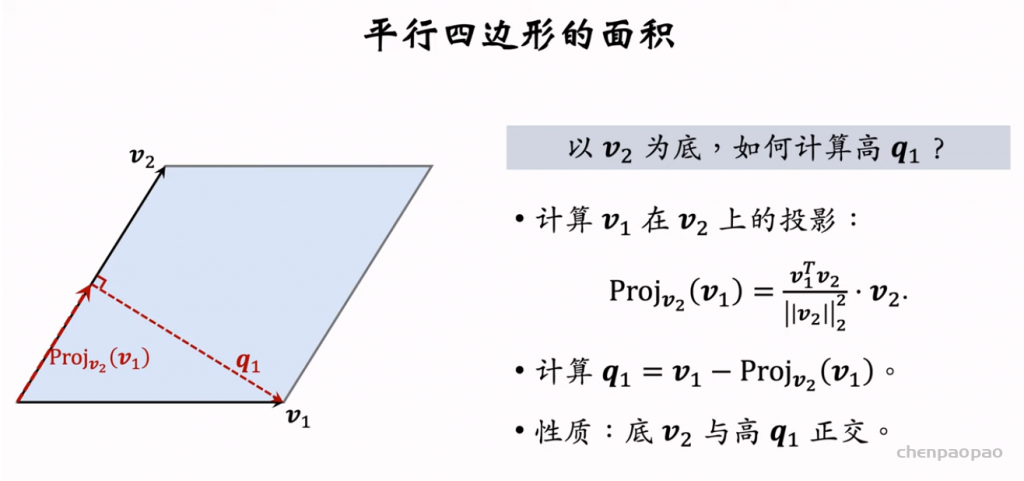
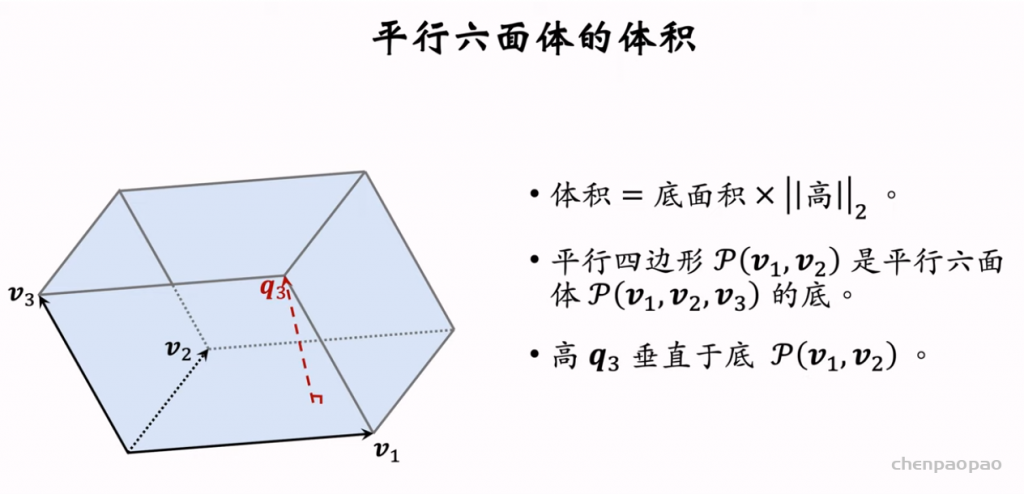
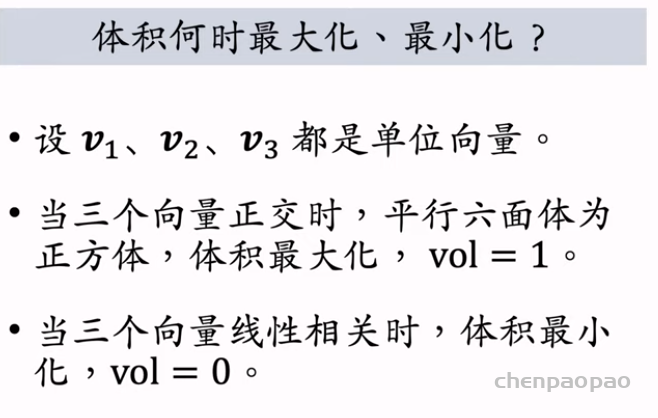
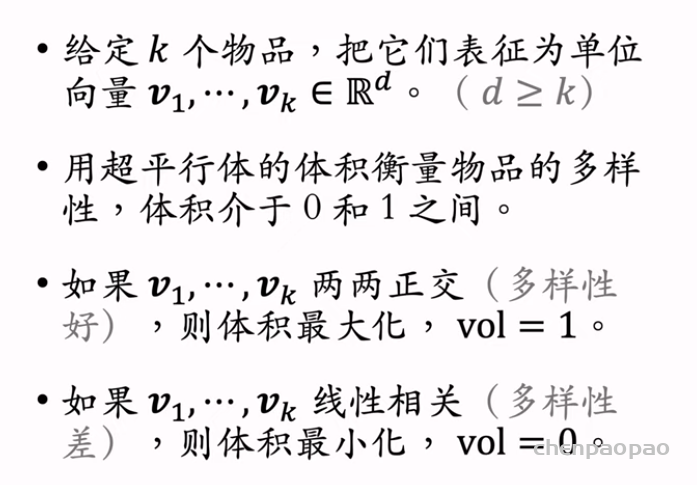
行列式等价于体积,因此用行列式的值来衡量物品的多样性。
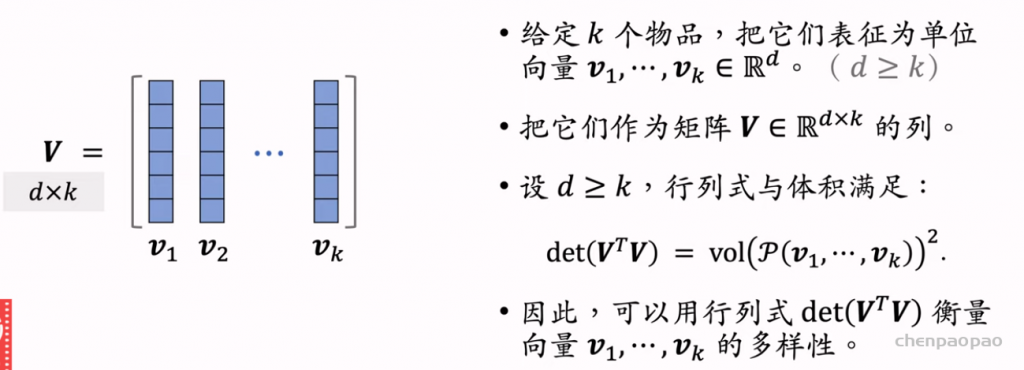
DPP及其再推荐系统重排中的应用。求解DPP是比较困难的,需要计算行列式很多次,而计算行列式需要矩阵分解,代价很大。这节课介绍Hulu论文中的算法,可以用较小的代价求解DPP。 参考文献: Chen et al. Fast greedy map inference for determinantal point process to improve recommendation diversity. In NIPS, 2018.
行列式等价于体积,因此用行列式的值来衡量物品的多样性。
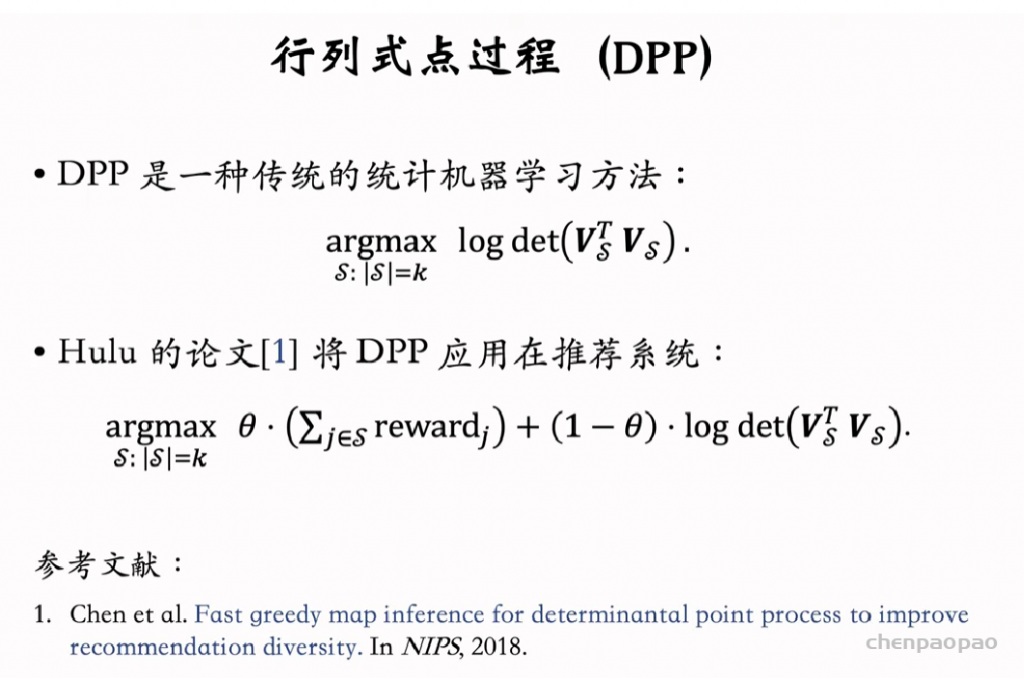
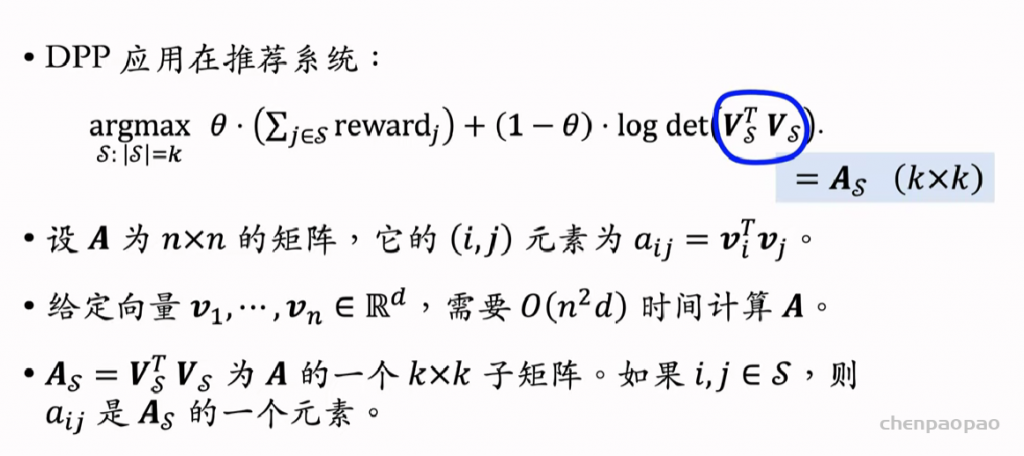
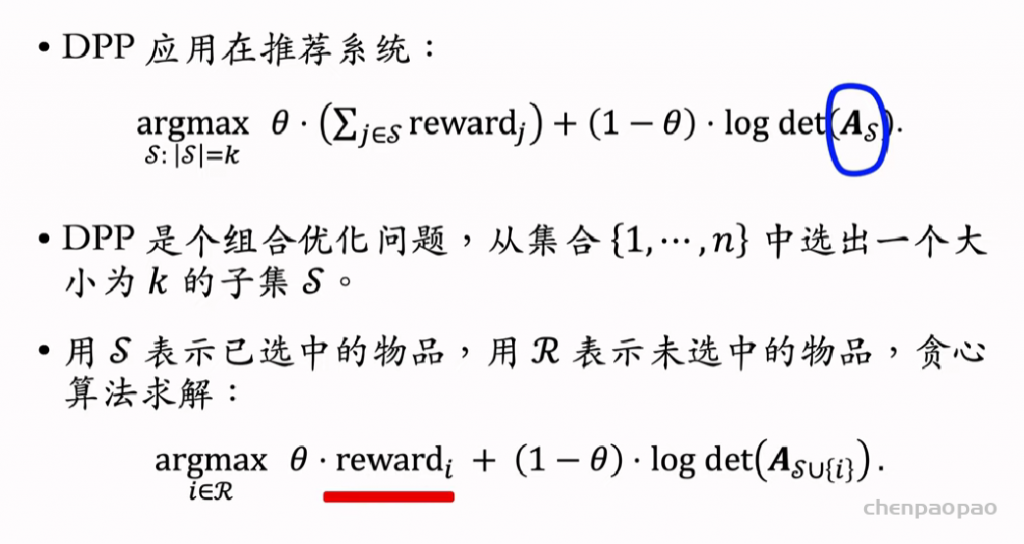
求解DPP:
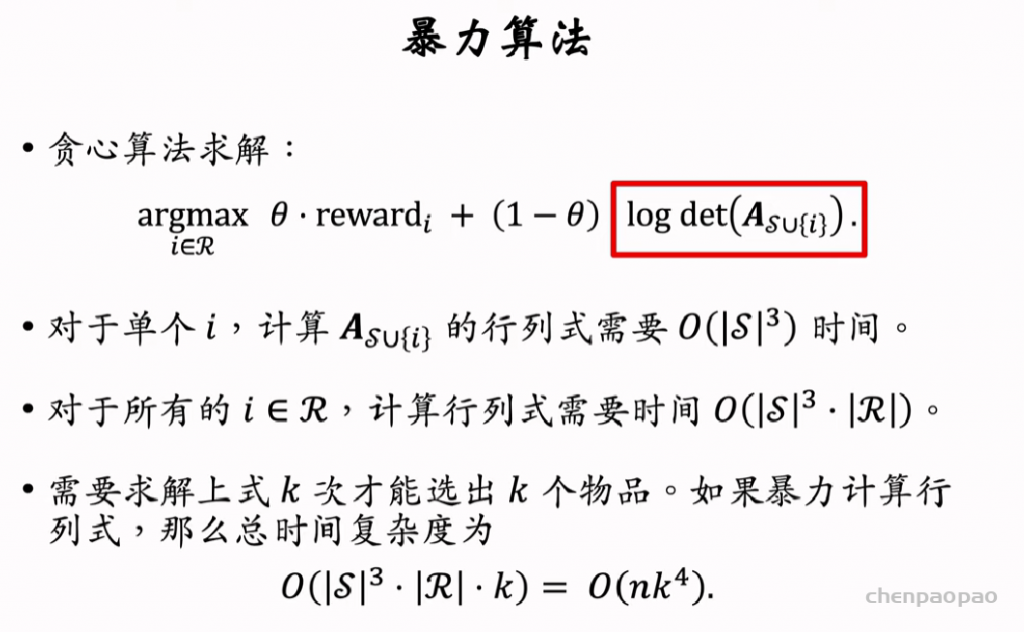
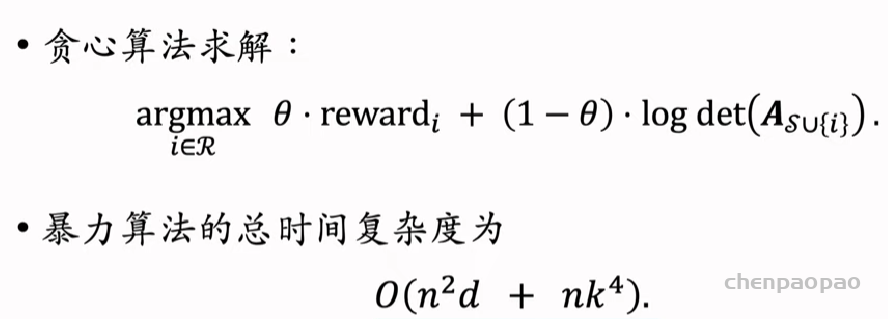
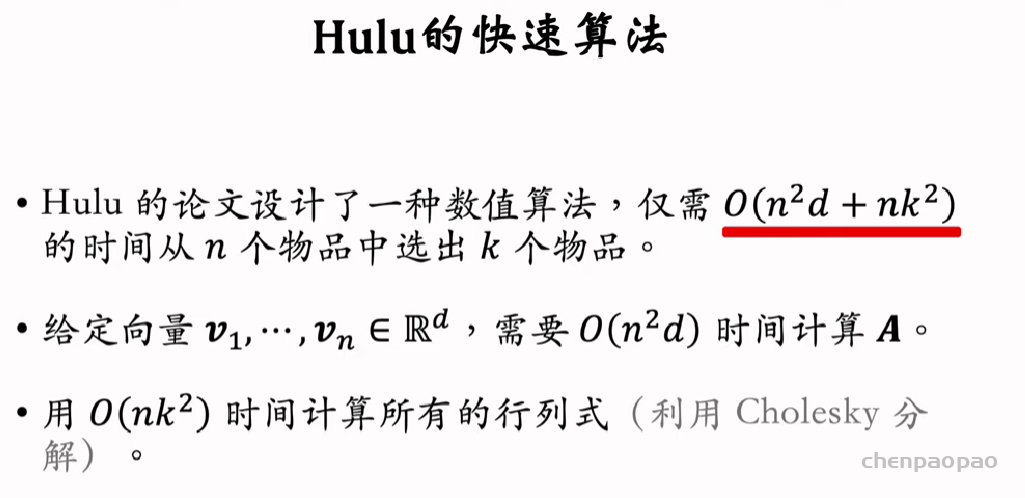
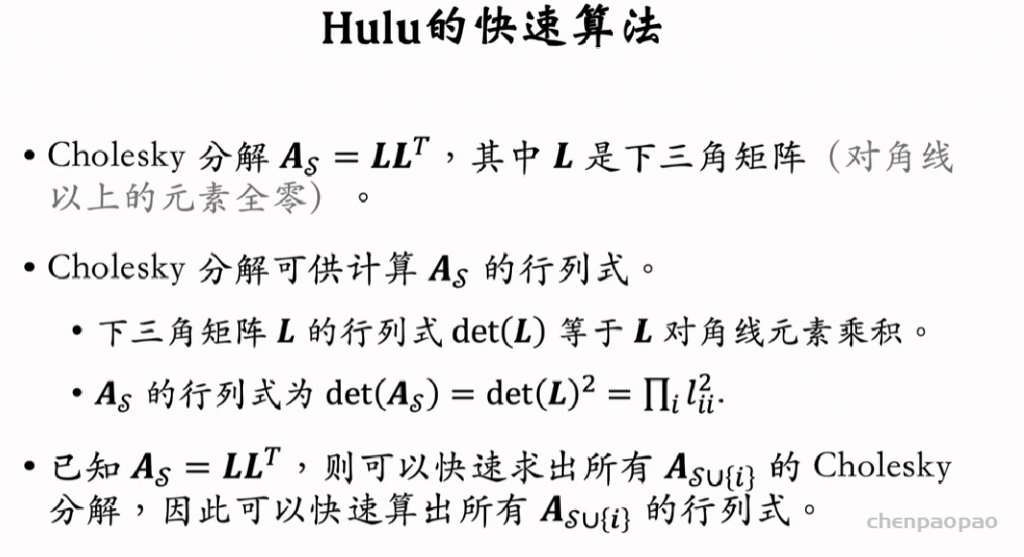
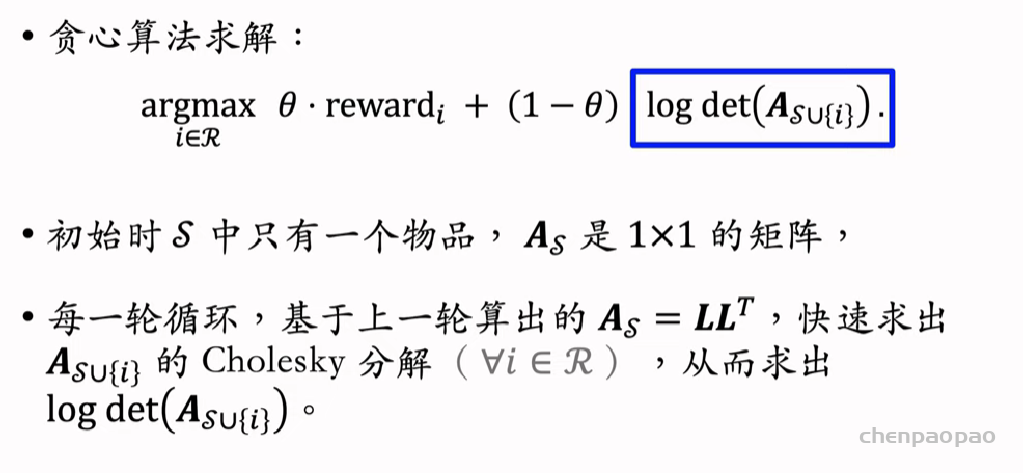
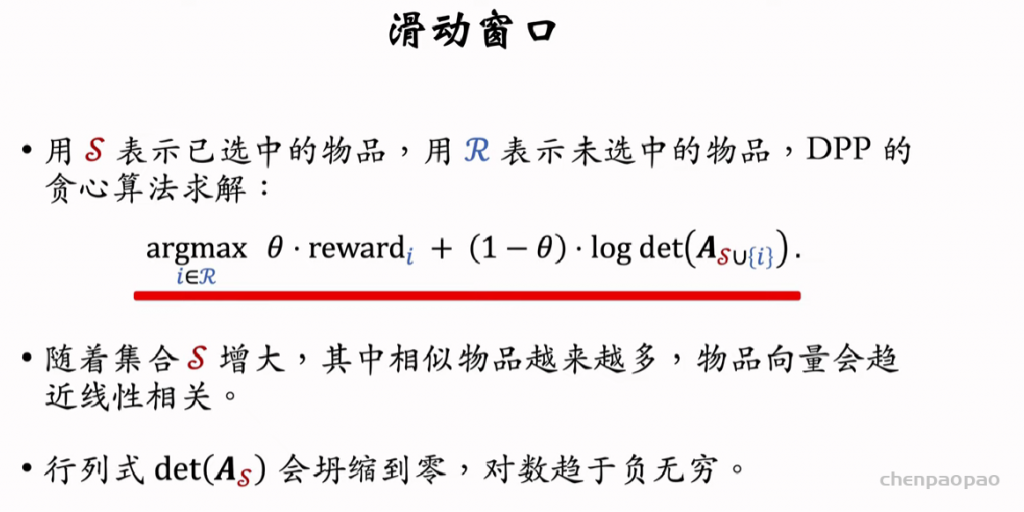
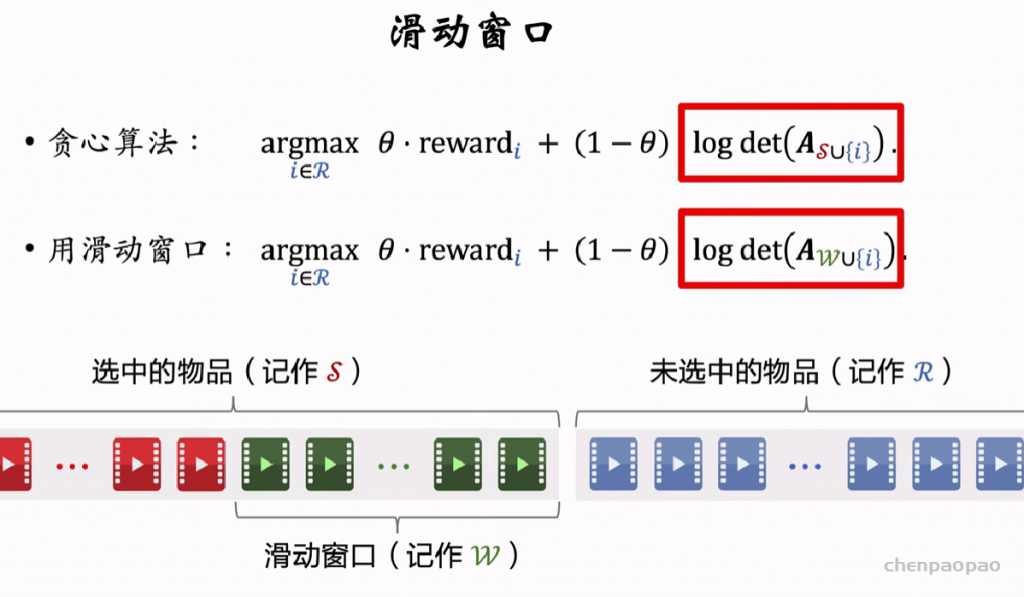
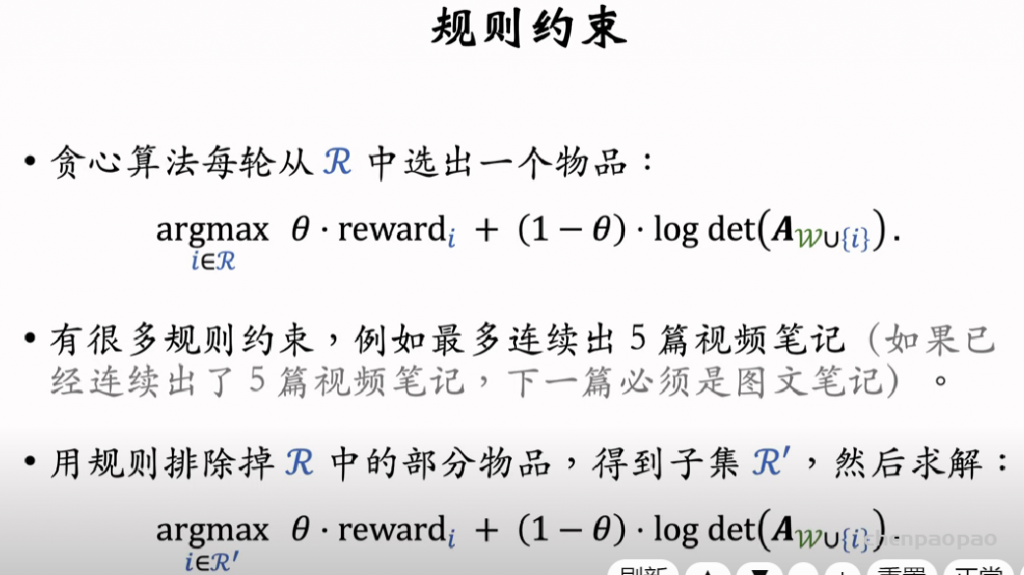